Prevalent and dynamic binding of the cell cycle checkpoint kinase Rad53 to gene promoters
Abstract
Replication of the genome must be coordinated with gene transcription and cellular metabolism, especially following replication stress in the presence of limiting deoxyribonucleotides. The Saccharomyces cerevisiae Rad53 (CHEK2 in mammals) checkpoint kinase plays a major role in cellular responses to DNA replication stress. Cell cycle regulated, genome-wide binding of Rad53 to chromatin was examined. Under replication stress, the kinase bound to sites of active DNA replication initiation and fork progression, but unexpectedly to the promoters of about 20% of genes encoding proteins involved in multiple cellular functions. Rad53 promoter binding correlated with changes in expression of a subset of genes. Rad53 promoter binding to certain genes was influenced by sequence-specific transcription factors and less by checkpoint signaling. However, in checkpoint mutants, untimely activation of late-replicating origins reduces the transcription of nearby genes, with concomitant localization of Rad53 to their gene bodies. We suggest that the Rad53 checkpoint kinase coordinates genome-wide replication and transcription under replication stress conditions.
Editor's evaluation
The unexpected localization of a cell cycle checkpoint kinase, Rad53, to promoters in response to replication stress suggests that Rad53 may help coordinate transcription in response to disrupted replication. This work will be of interest to those interested in the interplay between genome stability and gene expression.
https://doi.org/10.7554/eLife.84320.sa0Introduction
Rad53, the homolog of the mammalian CHEK2 kinase present in the budding yeast Saccharomyces cerevisiae, is an essential kinase involved in a multitude of cellular processes. The most well-established function of Rad53 is its role as an effector kinase in response to various sources of DNA damage. To maintain genome stability during S-phase, a DNA replication checkpoint (DRC) is activated in response to replication stress via the sensor kinase Mec1 (the mammalian ATM/ATR), the replication fork protein Mrc1 (Claspin in mammals), and other fork proteins (Lanz et al., 2019; Osborn and Elledge, 2003; Pardo et al., 2017; Paulovich and Hartwell, 1995; Saldivar et al., 2017). A second DNA damage checkpoint mediated by Rad9 (TP53BP1 in mammals) responds to double strand DNA breaks. Both branches converge on the effector kinase Rad53, which triggers a wide range of downstream events, including slowing or halting cell cycle progression, transiently preventing initiation of DNA replication at origins that have not yet replicated, activating DNA repair pathways and elevating the synthesis of deoxyribonucleoside triphosphates (dNTPs) by upregulating genes encoding the ribonucleotide reductase (RNR). The checkpoint signaling also promotes widespread changes in gene expression (Jaehnig et al., 2013; Pardo et al., 2017).
Unlike most of the checkpoint genes, both Mec1 and Rad53 kinases are essential for cell viability in unperturbed cells. This can be partly explained by their role in regulating dNTP pools (Desany et al., 1998; Forey et al., 2020; Zhao et al., 2000). However, under the bypass conditions in cells without Sml1, the inhibitor of the RNR enzyme, cells lacking either kinases are viable. Yet, it is important to note that these kinase-null mutants are extremely sick and sensitive to various types of exogenous stress. Furthermore, lacking Rad53 causes a more severe defect than lacking Mec1, implying that Rad53 has activities beyond checkpoint signaling. Consistent with this suggestion, the kinase-deficient mutant rad53K227A lacks checkpoint function but retains growth-associated activity and an additional rad53 mutation was found that is checkpoint proficient but supports cell growth poorly (Gunjan and Verreault, 2003; Hoch et al., 2013; Holzen and Sclafani, 2010; Pellicioli et al., 1999).
Rad53 is central to the transcriptional response to DNA damage. It is well established that the Dun1 protein kinase acts downstream of Rad53 to phosphorylate and inactivate the transcriptional repressor Rfx1/Crt1 and thereby upregulate target genes (Huang et al., 1998), including RNR2, RNR3, and RNR4, encoding subunits of RNR. However, the induced expression of RNR1, which encodes the major isoform of the RNR large subunit, is not controlled by the Rfx1 repressor, but by Ixr1 binding to the RNR1 promoter upon genotoxic stress (Tsaponina et al., 2011). This Ixr1-dependent regulation of RNR1 is independent of Dun1 but requires Rad53. Another Rad53-dependent, Dun1-independent regulation of RNR1 involves dissociation of the Nrm1 repressor from MBF following Nrm1 phosphorylation by Rad53 (Travesa et al., 2012). Thus, the Rad53-dependent transcription control is complex, but not yet fully understood.
In addition to upregulating the dNTP pools, defects in cells lacking Rad53 can be suppressed by manipulating factors functioning in transcription regulation, cell wall maintenance, proteolysis, and cell cycle control (Desany et al., 1998; Manfrini et al., 2012). Moreover, Rad53 kinase targets and interaction partners found in biochemical and proteomic studies suggest that the kinase is pleiotropic (Gunjan and Verreault, 2003; Jaehnig et al., 2013; Lao et al., 2018; Smolka et al., 2007; Smolka et al., 2006).
In this study, while investigating the response of yeast cells to replication stresses caused by depletion of dNTPs, we found that Rad53 localized to more than 20% of gene promoters in the S. cerevisiae genome, suggesting a multifaceted role in coordinating stress responses. Furthermore, we provide evidence that in the absence of the DRC checkpoint pathway, untimely activation of replication from late origins can negatively affect transcription activity of nearby genes.
Results
Rad53 is recruited to genomic loci other than replication origins in proliferating yeast cells
Previous studies focusing on specific genomic features, mainly subsets of DNA replication origins, have detected Rad53 binding to replication origins and replication forks (Can et al., 2019; Dohrmann and Sclafani, 2006). However, given the evidence that Rad53 has functions beyond the control of DNA replication stress, it is possible that Rad53 also functions at additional genomic loci. We therefore investigated the genome-wide distribution of Rad53 by chromatin immunoprecipitation and deep sequencing (ChIP-seq) in proliferating yeast cells (Behrouzi et al., 2016). Since the sml1 null mutation (sml1Δ) allows cells to bypass the requirement for Rad53, or its upstream kinase Mec1, for growth, we also performed ChIP-seq in the sml1Δ mutant and the rad53 null (rad53Δ sml1Δ) as controls for antibody specificity (Figure 1).
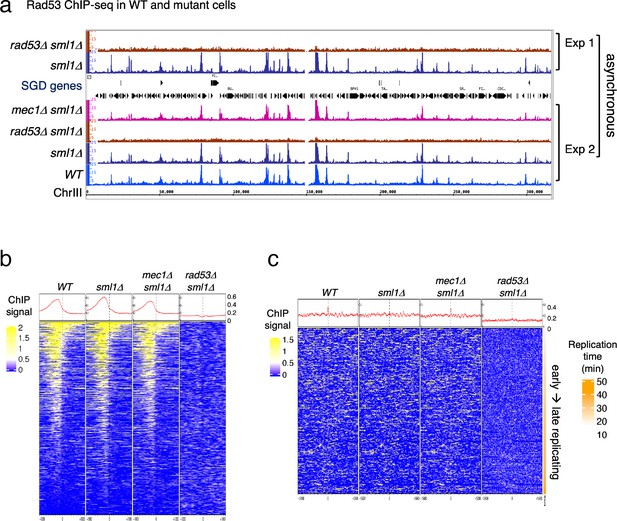
Rad53 is recruited to genomic loci other than replication origins in proliferating yeast cells.
(a) Coverage tracks of Rad53 ChIP-seq signals in WT, sml1Δ, rad53Δsml1Δ, and mec1Δ sml1Δ for chromosome III. Asynchronous yeast cultures were processed for ChIP-seq analysis for distribution of Rad53 at genomic loci. The results from two independent experiments are presented. Experiment 1 compares only sml1Δ, rad53Δsml1Δ. (b) Heatmaps and average signals of Rad53 across 2-kb intervals centered on transcription start sites (TSSs) for proliferating WT, sml1Δ, rad53Δsml1Δ, and mec1Δ sml1Δ cells. (c) Heatmaps of ChIP-seq signals across 30-kb intervals centered on all origins annotated in OriDB database (Siow et al., 2012).
Visual inspection of the ChIP-seq peaks from normalized coverage tracks suggested that Rad53 bound to sites throughout the genome (Figure 1a). Rad53 binding to the same sites also occurred in the sml1Δ mutant and the mec1Δ sml1Δ mutant, but was absent in the rad53Δ sml1Δ mutant. Computational analysis was performed to generate heatmaps of Rad53 ChIP-seq signal across 2-kb intervals centered on transcription start sites (TSSs) (Figure 1b). The average Rad53 ChIP-seq signal on top of the heatmap show that Rad53 was most concentrated within 500-bp upstream of TSSs in WT, sml1Δ, and mec1Δ sml1Δ mutants. A smaller fraction of genes also have signal within the gene bodies. No Rad53 signal beyond background was detected at TSSs in the rad53Δ sml1Δ cells, demonstrating antibody specificity. Analysis by calculating empirical p values for the ChIP-seq signals 500-bp upstream of TSS found that, out of 6604 genes, 1464, 1983, and 1293 in WT, sml1Δ, and mec1Δ sml1Δ, respectively, exhibited significant Rad53 signal at this region. This represents ~20% or greater of all gene promoters.
Heatmap analysis was also performed on Rad53 ChIP-seq signal across 30-kb intervals centered on all origins annotated in the OriDB database (Siow et al., 2012). The average signal across the whole region was higher in WT, sml1Δ, and mec1Δ sml1Δ than in rad53Δ sml1Δ after normalization (Figure 1c). However, the signal was not concentrated at the origins. Since these ChIP-seq datasets are from asynchronous cells, this finding is consistent with the idea that recruitment of Rad53 to replication origins is cell cycle regulated rather than constant binding to origins.
Binding of Rad53 to upstream TSS changes with cell cycle stages
To gain insight into the dynamics of Rad53 recruitment to genomic loci such as promoters and replication origins, Rad53 ChIP-seq was analyzed in samples from synchronized cell cultures. Cells were arrested in G1 using α-factor and then released into media containing hydroxyurea (HU) to induce replication stress caused by limiting dNTPs. Three stages of synchronous cell cultures were collected and referred to as G1 (for cells arrested in G1), HU45 and HU90 (for cells released from G1 into HU for 45 and 90 min, respectively). These cell samples were then processed for ChIP-seq analysis. Heatmaps of the Rad53 signals at 2-kb intervals centered on all TSSs show a trend of increasing Rad53 binding as cells progress from G1-phase into HU45 or HU90 (Figure 2a), concomitant with increased levels of Rad53 protein in cells treated with HU (Figure 2—figure supplement 1). The increase in Rad53 parallels entry into S-phase, as measured by Orc6 phosphorylation (Figure 2—figure supplement 1a). Similar to the data from asynchronous cell samples, Rad53 signals were most concentrated upstream of TSSs (Figure 2a, left panel). The ChIP-seq signals using antibodies against γ-H2A, which like Rad53 is a target of the sensor kinase Mec1, were not enriched upstream of TSSs (Figure 2a, right panel). In fact, the signals for γ-H2A were lower immediately upstream of TSSs than the surrounding regions, consistent with promoters being histone-free regions.
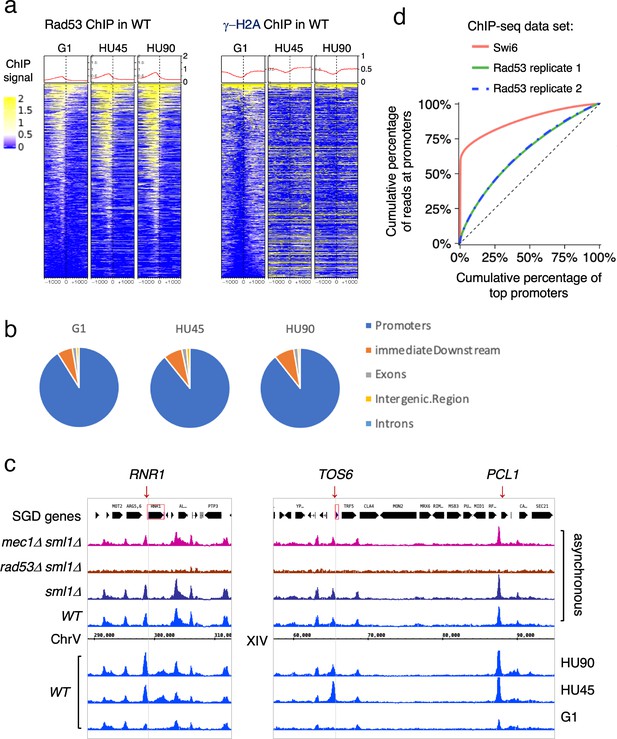
Rad53 is recruited to transcription start site (TSS) and the binding changes with the cell cycle stage.
Cells were synchronized in G1 phase and released into YPD containing 0.2M hydroxyurea (HU) for 45 and 90min (HU45 and HU90, respectively). (a) Heatmaps and average signals of Rad53 and γ-H2A ChIP-seq signals across 2-kb intervals centered on TSSs for WT cells at stages of G1, HU45, and HU90. (b) Distribution of aggregated peak numbers around TSS using merged Rad53 ChIP-seq peaks from all three stages (G1, HU45, and HU90). Pie charts showing the distribution of Rad53 ChIP-seq peaks in relation to gene features. (c) Rad53 ChIP-seq profiles near RNR1, PCL1, and TOS6 genes in proliferating WT, sml1Δ, rad53Δ sml1Δ, and mec1Δ sml1Δ cells, and WT cells at stages of G1, HU45, and HU90. (d) Lorenz curves for ChIP-seq read counts of Swi6 and Rad53 mapped to gene promoters showing inequality for promoter binding.
Further analysis showed that >85% of the Rad53 ChIP-seq peaks overlap with gene promoters (defined as 500-bp upstream and 50-bp downstream of TSS) at all stages investigated. The distribution of aggregated peak numbers around TSSs showed highest count numbers immediately upstream of the TSS (Figure 2b). By visual inspection of normalized ChIP-seq coverage tracks, we noticed that signals at promoters of genes such as RNR1, PCL1, and TOS6 varied depending on the cell cycle stage (Figure 2c, bottom three tracks for WT at stages of G1, HU45, and HU90) while signals at neighboring gene promoters remained largely constant. Thus, the recruitment of Rad53 to a subset of gene promoters is regulated. Because RNR1, PCL1, and TOS6 are known targets of the cell cycle regulator SBF, a sequence-specific transcription factor composed of subunits Swi4 and Swi6, we compared Rad53 ChIP-seq data and a previous ChIP-seq dataset of Swi6 (Park et al., 2013). As indicators for the gene specificity of protein binding, the Gini indices were computed from Lorenz curves of ChIP-seq data for Swi6 and two of our Rad53 replicates (Figure 2d), being 0.763, 0.2918, and 0.2982, respectively. Rad53 has a coverage for many promoters while Swi6 shows substantially high coverage only for a limited number of promoters. Thus, it is likely Rad53 would effect a wider range of genes than the Swi6 regulatory network.
Previous studies have found that under certain conditions, regions of the genome are promiscuously present in ChIP-seq studies independent of the antibody used (Park et al., 2013; Teytelman et al., 2013). These regions were termed hyper-ChIPable regions and were found enriched for sequences in and around gene bodies of highly expressed genes. We therefore examined whether these regions were promiscuously present under our conditions. Analysis of ChIP-seq data for Rad53 and γ-H2A with or without the hyper-ChIPable regions observed by Teytelman et al. and Park et al. did not alter the pattern of Rad53 binding to TSSs (Figure 2—figure supplement 2a–d). When we specifically examined the pattern of Rad53 ChIP-seq signals around TSSs of the 296 genes associated with those hyper-ChIPable sequences, we observed not only localization to TSSs, but also enrichment to the gene bodies as previously reported (Park et al., 2013; Teytelman et al., 2013). This pattern is distinct from the pattern of promoter localization (compare Figure 2—figure supplement 2d, e). Furthermore, KEGG analysis of the genes enriched in the studies by Teytelman et al. and Park et al. showed predominantly genes encoding snoRNAs and tRNAs, genes we did not find in the promoter binding for Rad53 (Figure 2—figure supplement 2f). Finally, we did not observe any enrichment at gene promoters when the RAD53 gene was deleted from the strain (Figure 1a, b) or when anti-γ-H2A antibodies were used (Figure 2a). Thus, we suggest that the Rad53 binding observed here is not the same as the promiscuous, non-specific enrichment of hyper-ChIPable regions reported previously. Moreover, Rad53 binding to a subset of promoters is transcription factor dependent (see below).
Rad53 is recruited to sites of DNA synthesis independent of checkpoint signaling
Since previous studies reported localization of Rad53 to replication origins (Can et al., 2019; Dohrmann and Sclafani, 2006), we also performed heatmap analysis of ChIP-seq signal around replication origins for Rad53, γ-H2A, and Cdc45, a component of active helicase complex and hence the marker for active replication forks (Figure 2—figure supplement 3). The 30-kb window for the heatmap around origins was chosen based on our prior knowledge that the extent of DNA synthesis in WT cells under similar growth conditions at HU90 was about 10 kb (Sheu et al., 2014). In WT cells, the Rad53 signal was present at regions associated with early firing origins but not with late firing origins that are inactive due to inhibition by the HU-induced checkpoint. However, Rad53 signal was also present at late origins in the kinase-deficient rad53K227A and mrc1Δ mutants, both of which allow activation of late origins as a result of the checkpoint defect. Thus, Rad53 was recruited only to replication forks associated with activated origins and the pattern was similar to that of Cdc45. Surprisingly, Rad53 binding to replication forks did not require Mrc1 or its own kinase activity, suggesting checkpoint-independent recruitment of Rad53 to sites of DNA synthesis.
Interestingly, γ-H2A was observed at genomic regions surrounding the very late origins in G1-phase in both WT and mutants (Figure 2—figure supplement 3b). It is possible that these γ-H2A signals reflect a low level of ssDNA gaps at these late-replicating regions that were tolerated and carried over from the previous cell cycle, similar to unrepaired post-replication gaps resulting from low level of UV irradiation found in S. pombe G2-phase (Callegari and Kelly, 2006).
Identification of genes with differential binding of Rad53 at promoters
Rad53 promoter binding was temporally dynamic in a subset of genes, suggesting regulation by cell cycle progression or DNA replication stress. To identify genes with differential or dynamic binding of Rad53 at their promoters (DB genes), we applied residual analysis. The read count difference was investigated for the promoter regions (500-bp window upstream of TSSs) of all genes for Rad53 ChIP-seq. The comparison was done between stages G1 and HU45 of WT samples from two independent experiments (termed CP and TF, Figure 3a). Since each dataset had two biological replicates from stages G1 and HU45, we extracted the top 1000 genes that displayed dynamic binding from the aggregated read coverage and called these genes the top DB genes (Figure 3a). Among the 1000 top DB genes from each set of comparison, 435 genes were identified in both sets (435 top DB overlap).
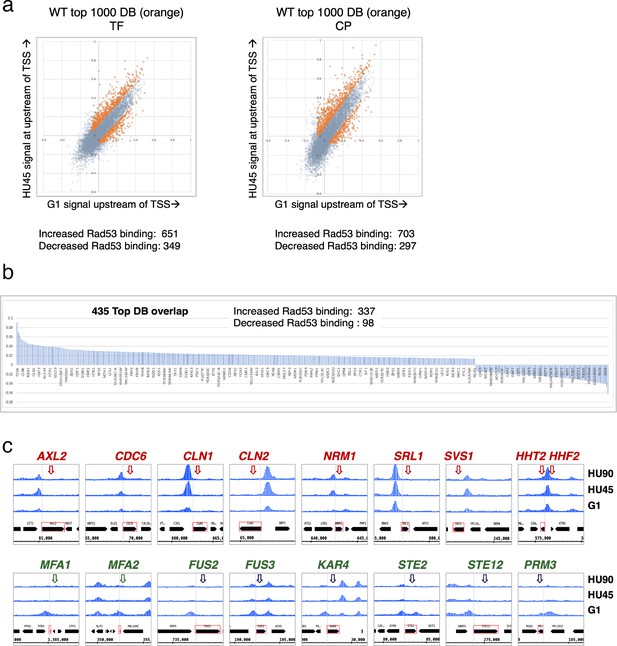
Identification of genes with Rad53-binding changes at the promoters.
(a) Scatter plots compare the signals in G1 (x-axis) and HU45 (y-axis) at 500-bp intervals upstream of transcription start sites (TSSs) for all genes in WT datasets from two independent experiments, TF and CP. Orange dots indicated the 1000 genes with highest binding changes (top 1000 DB) and satisfying the filter of minimal signal of −0.075 (Maximal = 1). The Venn diagram to the right illustrates the identification of 435 genes that are found in both experiments (435 top DB overlap). (b) Binding changes (y-axis: residuals from analysis of experiment TF) for 435 top DB overlap. (c) Examples of coverage tracks for selected genes show Rad53 signal changes at the indicated gene promoters from G1 to HU45 and HU90.
Overall, during the G1- to S-phase transition (HU45), there are more genes with increased Rad53 promoter binding than those with decreased binding: within the list of 435 genes, 337 show increased Rad53 binding at their promoters, while 98 show decreased binding (Figure 3b). These genes include those involved in cell cycle progression (e.g., genes encoding cyclins and regulators of DNA replication) and cell growth (e.g., cell wall maintenance and mating response). Figure 3c shows Rad53 dynamic binding, either up or down, at the promoters of representative genes as cells transitioned from G1 phase to HU45 and HU90 time points.
The relationship between Rad53 promoter binding and gene expression
To gain insight into the relationship between Rad53 promoter recruitment and gene expression, RNA-seq analysis was performed under the same growth conditions used for the ChIP-seq experiments. RNA-seq replicates of datasets from four yeast strains (WT, rad9Δ, rad53K227A, and mrc1Δ), each with three stages (G1, HU45, and HU90) were analyzed using rank data analysis (Figure 4a). In the hierarchical clustering, cell cycle stage contributes more to similarities in expression than the genotype. In particular, the expression profiles in G1 were very similar among all strains. In HU45, however, two subgroups clustered by genotype were evident: rad9Δ clustered with WT, consistent with Rad9 having no role in the DRC checkpoint branch, while rad53K227A and mrc1Δ clustered together, consistent with Rad53 and Mrc1 functioning together in the DRC response to HU stress.
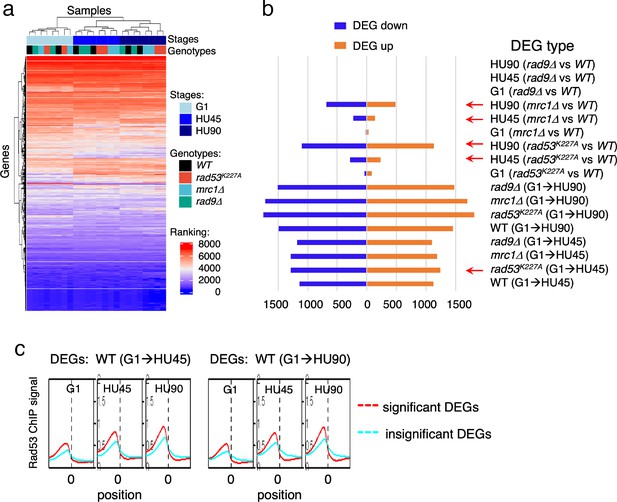
Gene expression changes in WT and checkpoint mutants under stress and the tendency of higher Rad53 binding at promoter of genes with significant differential expression.
WT, rad9Δ, rad53K227A, and mrc1Δ cells were synchronized in G1-phase and released into YPD containing 0.2M hydroxyurea (HU). Cells at stages of G1, HU45, and HU90 were collected and processed for RNA-seq analysis. (a) Rank data analysis of RNA-seq samples. (b) Bar graph summarizing on the x-axis the number of genes that show statistically significant differential expression (DEGs). The types of pairwise comparison are indicated to the right. Blue bars, downregulated DEGs. Orange bars, upregulated DEGs. (c) Average Rad53 ChIP-seq signal across 2-kb intervals centered on at transcription start site (TSS) for statistically significant DEGs (red) and the insignificant (cyan). Left panel shows the result of DEGs from comparison of G1 and HU45 datasets of WT (DEGs: WT(G1 → HU45)) and right panel shows DEGs from comparison of G1 and HU90 datasets (DEGs: WT(G1 → HU90)).
Pairwise comparisons of G1 to HU45 samples showed that, in both WT and rad9Δ cells, ~2300 genes exhibited significant expression changes (differentially expressed genes, DEGs; Figure 4b). The number of DEGs increased to ~3000 when comparing G1 to HU90. Moreover, in both rad53K227A and mrc1Δ mutants, ~2500 DEGs were detected from G1 to HU45, which increased to >3400 in G1 to HU90. The response to cell cycle stage was largely equally distributed between up- and downregulation. Comparison of WT to rad53K227A in the HU45 and HU90 conditions found 517 and 2234 DEGs, respectively, while comparison of WT to mrc1Δ revealed 370 and 1166 DEGs in HU45 and HU90. A WT and rad9Δ comparison at all stages showed only five DEGs, including the deleted RAD9 gene and its marker cassette HIS3. Thus, Rad9 did not contribute much to gene expression changes under HU stress.
Based on ChIP-seq data, the average Rad53-binding upstream of TSSs was higher in the significant DEGs than in the insignificant, when comparing G1 to HU45 or to HU90 of the WT datasets (Figure 4c and Figure 4—figure supplement 1a). These results suggested that Rad53 may play a role in the control of gene expression at a subset of expressed genes. Similar observations were also apparent when comparing stage-dependent samples in the checkpoint mutants (Figure 4—figure supplement 1b, c), demonstrating that the functions of Rad53 at gene promoters were not solely checkpoint dependent. Therefore, we performed gene coexpression analysis based on association in the coexpression network (Lee et al., 2020). Coexpression analysis of significant DEGs in WT(G1 → HU45) yielded 10 coexpression clusters (Figure 5a). Dynamic Rad53 binding at promoter regions occurred in most clusters (Figure 5b), with significantly enriched GO functions including cell cycle regulation, mating response, proteolysis, transport, oxidation–reduction process, and organic acid metabolism (Figure 5a). Within the 435 top DB overlap gene group (Figure 3b), 236 genes were also detected as DEGs (236 DB/DEGs) in the WT(G1 → HU45) comparison. The association between binding changes and expression changes was significant (Figure 5d, left panel; Fisher’s exact test p < 0.0001). Plots of Rad53-binding changes against gene expression changes of these 236 DB/DEGs revealed a moderate positive correlation between Rad53-binding change and gene expression change, with a Spearman r = 0.5918 (Figure 5c, left panel). Among this group, 51 out of 54 genes with decreased Rad53 signal were downregulated in mRNA levels, whereas genes with increased Rad53 signals partitioned into both up- and downregulation (108 and 74, respectively). Further break down of the 236 DB/DEGs group by coexpression clusters revealed that genes in clusters 1 and 7 exhibited the most significant positive correlation between Rad53-binding and gene expression changes (Figure 5c, r = 0.5697 (p < 0.000001) and r = 0.6979 (p < 0.000037), respectively). Thus, specific subsets of DEGs in the shift from G1 → HU exhibited correlations between a change in gene expression and Rad53 promoter binding.
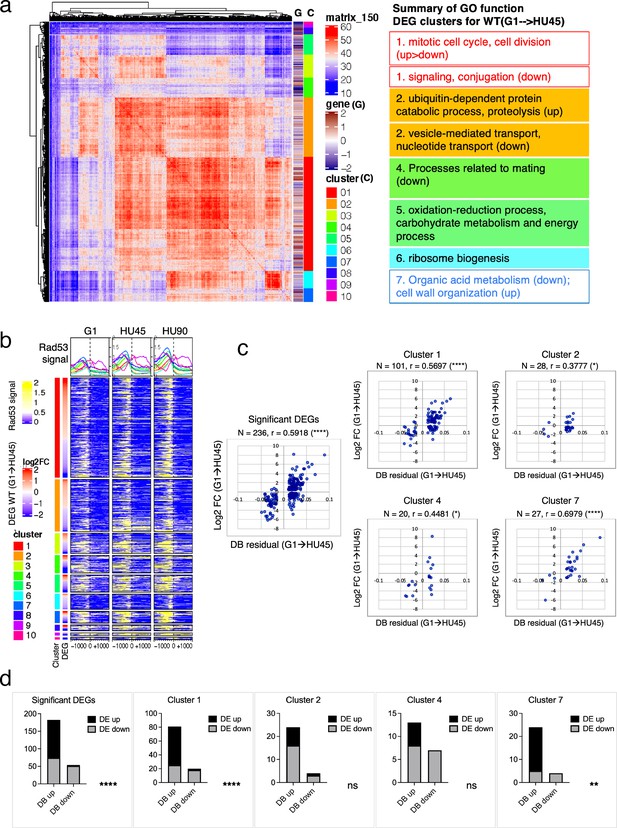
Correlation between differential binding of Rad53 at promoter and differential gene expression (DEG).
(a) Coexpression cluster matrix for significant DEGs in WT(G1 → HU45). Cluster (C): color codes for DEG clusters. Gene (G): level of differential expression in log2FC. (b) Heatmaps of Rad53 ChIP-seq signal across 2-kb intervals centered on transcription start site (TSS) parsed by the DEG clusters in (a). Genes within each cluster are arranged in descending order according to the differential expression level (i.e., log2FC). (c) Scatter plots of Rad53-binding changes at the promoter against expression changes for the 236 significant DEGs in the 435 top DB overlap group (leftmost panel) and subgroups in clusters 1, 2, 4, and 7. Spearman’s correlation r is indicated on top of each plot (****p < 0.0001; *p < 0.05). N, number of genes in the group analyzed. (d) Result of Fisher’s exact test for association between binding changes (DB) and expression changes (DE) for groups presented in (c). ****p < 0.0001; **p < 0.01; ns, not significant. Examples of genes in the cluster are shown below the plot.
Checkpoint mutants cause downregulation of gene expression near promiscuously active late origins
We further inspected the localization of Rad53 in a subset of DEG clusters from the HU45 (mrc1Δ vs. WT) comparison (Figure 6a) and the HU45 (rad53K227A vs. WT) comparison (Figure 6—figure supplement 1a). In these analyses, we noticed a characteristic pattern, in which downregulated genes tended to have a strong Rad53 signal not only upstream of the TSS, but a broad signal within gene bodies (Figure 6b and Figure 6—figure supplement 1b). This pattern was prominent in the mrc1Δ mutant in HU45 and further intensified in HU90. The gene body localization was also found more transiently in HU45 sample from rad53K227A cells. This signal pattern was not as prevalent in the WT HU45 and HU90 samples. Since Rad53 is also recruited to active origins and moves with the replication fork, we suspected that these gene body signals in the checkpoint mutants were caused by the promiscuous activation of near-by origins that are normally inactive in WT, creating conflicts between DNA replication and gene transcription. The transient nature of the Rad53 localization at gene bodies in this group of genes in the rad53K227A mutant is also consistent with the transient signal pattern at origins (Figure 2—figure supplement 3c). Thus, we investigated the relationship between these genes and their closest replication origins.
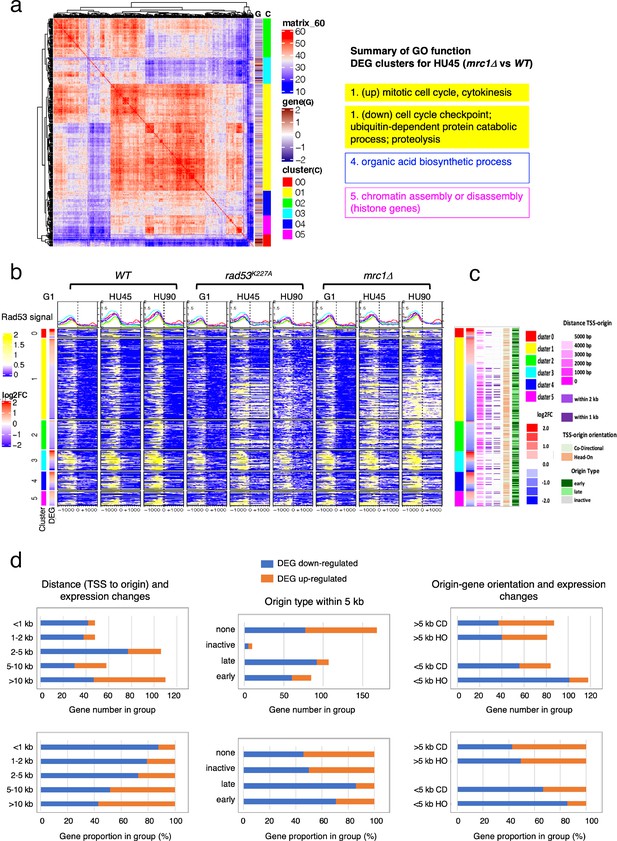
Origin-proximal differentially expressed genes (DEGs) are biased toward downregulation in the mrc1Δ mutant.
DEGs from HU45 (mrc1Δ vs. WT) comparison were analyzed. (a) Coexpression cluster matrix for significant DEGs. Cluster (C): color codes for DEG clusters. Gene (G): level of differential expression in log2FC. (b) Heatmaps of Rad53 ChIP-seq signal across 2-kb intervals centered on transcription start site (TSS) parsed by the DEG clusters in (a). ChIP-seq signal in WT, rad53K227A, and mrc1Δ mutant cells at stages G1, HU45, and HU90 are shown. (c) Summary of gene–origin relation for DEGs coexpression clusters. Distance between each TSS and its nearest origin center is indicated in pink gradient as well as light purple (<2kb) and dark purple (<1kb). Relative TSS-origin orientation and origin type are indicated. (d) Stacked bar charts presenting number (top panels) and proportion (bottom panels) of down- and upregulated DEGs as categorized by (1) TSS to origin distance (left panels), (2) closest origin type within 5kb of TSS (middle panels; none: no origins within 5kb of TSS), and (3) origin–gene orientation (right panels; CD: co-directional; HO: head-on).
The distance from the TSS of each gene to the nearest replication origins, the relative orientation of gene transcription to the origin (head-on [HO] or co-directional [CD]) and the origin type (early, late or inactive; see Definition of the origin types in Materials and methods) was determined and presented in the same order as in the heatmaps of DEG clusters shown in Figure 6b, c (Figure 6c). Overall, most of the downregulated genes in cluster 1 of this group were situated very close to active origins (<2 kb between origin center and TSS, light purple marks and <1 kb, dark purple marks). Interestingly, the pattern of marks for origin to TSS distance largely mirrored the patten of the Rad53 ChIP signal within the gene bodies (Figure 6b, c). This correlation pattern was not found in the WT ChIP heatmaps.
To explore the functional relationship between replication origins and genes, we summarized the ratio of up- and downregulated DEGs from the HU45 (mrc1Δ vs. WT) comparison in terms of the category of their relation to the closest origins (Figure 6d). In the first category, we parsed this DEG group according to the range of distance between TSS and closest origins (Figure 6d, left panels). Within this DEG group, genes situated 5 kb or more away from the closest active origins were similarly distributed between up- and downregulation of gene expression. However, for those genes that are closer to an active origin (<5 kb), the bias toward downregulated genes increased. For those genes situated less than 1 kb away from active origins, more than 80% were downregulated. In the second category, we grouped the DEGs according to the type origin the TSS was closest to. The DEGs that are more than 5 kb away from any annotated origins were labeled ‘none’ in origin type (Figure 6d, middle panels) and similarly distributed between up- and downregulation as expected from the above analysis. However, more downregulated genes were found when the nearby origins were active (Figure 6d, origin type late or early). The bias was stronger for genes that were close to late origins, which become active in HU when Mrc1 was absent (86% and 71% downregulated when genes were close to late and early origins, respectively, Fisher’s exact test p = 0.012). Because late origins and intermediate early origins were more active in the mrc1Δ mutant, it is possible that nearby gene expression was negatively affected by active DNA synthesis. In the third category, we examined the effect of relative gene–origin orientation. We found that the bias toward the downregulation was stronger when the nearby origin (<5 kb away) was in a HO orientation than in a CD orientation toward the gene (86% and 67% downregulated for HO and CD sets, respectively, Fisher’s exact test p = 0.0019) (Figure 6d, right panels). Thus, untimely activation of DNA replication origins in the checkpoint mutants affects gene expression concomitant with Rad53 binding to gene bodies.
Rad53-binding changes coincide with the changes in gene expression for targets of cell cycle regulators SBF and MBF
Two clusters from the gene coexpression analysis of DEGs in the WT(G1 → HU45) comparison showed a significant correlation between Rad53 binding and gene expression (Figure 5c, d, clusters 1 and 7). These two clusters contain genes that encode targets of SBF and MBF, key transcription factor complexes comprised of a shared regulatory subunit, Swi6 and the DNA-binding subunits Swi4 and Mbp1, respectively (Breeden, 2003). Their target genes include multiple G1- and S-phase cyclin genes, such as PCL1, CLN1, CLN2, CLB5, and CLB6. Evidence suggests that SBF and MBF are directly regulated by Rad53 kinase and Rad53 may regulate expression of targets of Msn4, Swi6, Swi4, and Mbp1 through Dun1-independent mechanisms (Jaehnig et al., 2013; Bastos de Oliveira et al., 2012; Sidorova and Breeden, 2003; Travesa et al., 2012). Thus, we analyzed the annotated targets of these transcription factors compiled in the Saccharomyces Genome Database (SGD; https://www.yeastgenome.org). Among the 81 genes that are candidate targets for both Swi4 and Swi6, 36 genes were found in the 236 DB/DEGs (Figures 3b and 5c) with an increase of frequency from 1.18% to 15.25% (p < 1E−15). Scatter plot comparisons of Rad53-binding and gene expression changes of these 36 genes show a clear positive correlation (Figure 7a, SBF top panel). Comparing with data from the checkpoint mutant samples, we found that most of these SBF target genes showed a similar profile of differential expression, from G1 to HU, in the rad9Δ mutant to that in WT (Figure 7b). However, in the mrc1Δ and rad53K227A mutants, a subgroup of genes, for example RNR1, SRL1, and YMR279C, exhibited different levels of change from that in WT (Figure 7b). We also found significant enrichment for targets of MBF (targets for both Mbp1 and Swi6 in SGD annotation) and transcription factor Msn4 among the 236 DB/DEGs group, as well as positive correlations between Rad53 promoter-binding and gene expression changes in these transcription factor targets (Figure 7a). Noticeably, there are 19 genes in these TF target group being both targets of SBF and MBF, and 12 out of 22 Msn4 targets that are also SBF targets.
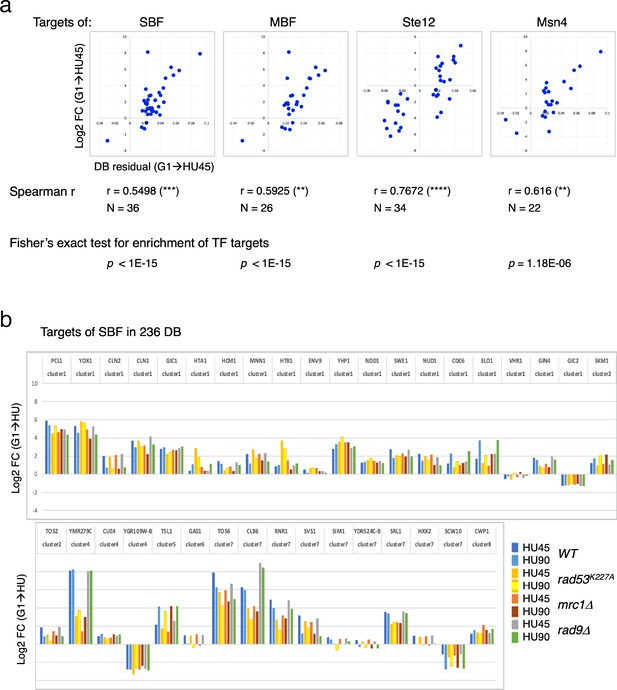
Differential binding of Rad53 at promoters and differential expression of target genes of SBF, MBF, Msn4, and Ste12.
(a) Top panels: scatter plots of binding changes (DB residual) and expression changes (log2FC) for targets of indicated transcription regulators that are in the 236 DB/differentially expressed genes (DEGs) group. Spearman’s correlation r is shown under each plot (****p < 0.0001; ***p < 0.001; **p < 0.01). N, number of genes in the group analyzed. Result of Fisher’s exact test for enrichment of each group of transcription regulator targets in the 236 DB/DEGs is also indicated below. (b) Profiles of differential expression in column graphs for each of the 36 SBF targets in the 236 DB/DEGs. Color-coded columns showing expression change (log2FC (G1 → HU45) and log2FC (G1 → HU90)) extracted from WT, rad53K227A, mrc1Δ, and rad9Δ datasets.
Many of the genes with decreased Rad53 binding at the promoters are mating response genes (Figure 3b, c, bottom panels). Therefore, the targets of Ste12, a key transcription factor activated by MAPK signaling to regulate genes involved in mating or pseudohyphal/invasive growth pathways were investigated. Of 183 potential targets of Ste12 annotated in SGD, 34 were in the 236 DB/DEGs group (Figures 5c and 7a). All the Ste12 targets that have decreased Rad53 binding were downregulated as cells entered S-phase. Moreover, 20 out of the 34 Ste12 targets in the top DB group showed increased Rad53 binding in HU and 11 of these 20 genes were also targets of SBF. Thus, regulation by SBF may be responsible for the correlation between increased Rad53 binding at the promoter and upregulation of these target genes.
SBF is a key factor for recruitment of Rad53 to the promoters of its target genes under replication stress condition
To determine the contribution of various transcription regulators in recruitment of Rad53 to gene promoters, we performed Rad53 ChIP-seq experiments for WT, ixr1Δ, swi4Δ, and swi6Δ mutants (experiment TF). The ixr1Δ mutant was also examined because a previous investigation found that Ixr1 binds to the RNR1 promoter upon genotoxic stress and mediates Dun1-independent RNR1 gene regulation that requires Rad53 (Tsaponina et al., 2011). In the scatter plot of the Rad53 signal upstream of TSSs in G1 versus HU45 from the WT dataset, SBF targets in the top DB (Figure 8a, orange/red diamonds) showed substantial deviation from the general trend (blue dots). In swi4Δ and swi6Δ mutants, the signal for all of these SBF targets collapsed toward the general trend (purple and light olive dots, swi6Δ and swi4Δ, respectively), suggesting that Rad53 signal changes at these genes depended on SBF. Analysis of Z-score distribution for Rad53 DB residual (G1 → HU45) also showed substantial deviation of SBF target genes from the rest of the genes in WT (Figure 8b), while in the swi4Δ and swi6Δ mutants, the deviation of the SBF targets was closer to other genes. Coverage tracks for Rad53 ChIP-seq signals showed that in the SBF mutants Rad53 binding was completely eliminated from the TOS6 (target of SBF 6) promoter while for PCL1 and YOX1, both targets of SBF, Rad53 binding did not increase in HU, in contrast to the pattern in WT (Figure 8c). Thus, SBF is important for the recruitment of Rad53 to the promoters of SBF target genes under replication stress. Interestingly, at the promoter of RNR3, the paralog of RNR1, Rad53 binding in the SBF mutants was low, even though RNR3 is known to be target of Rfx1 and not known as target of SBF or MBF. Thus, it is possible that, in addition to Rfx1, SBF also plays a role in regulation of RNR3 in response to replication stress.
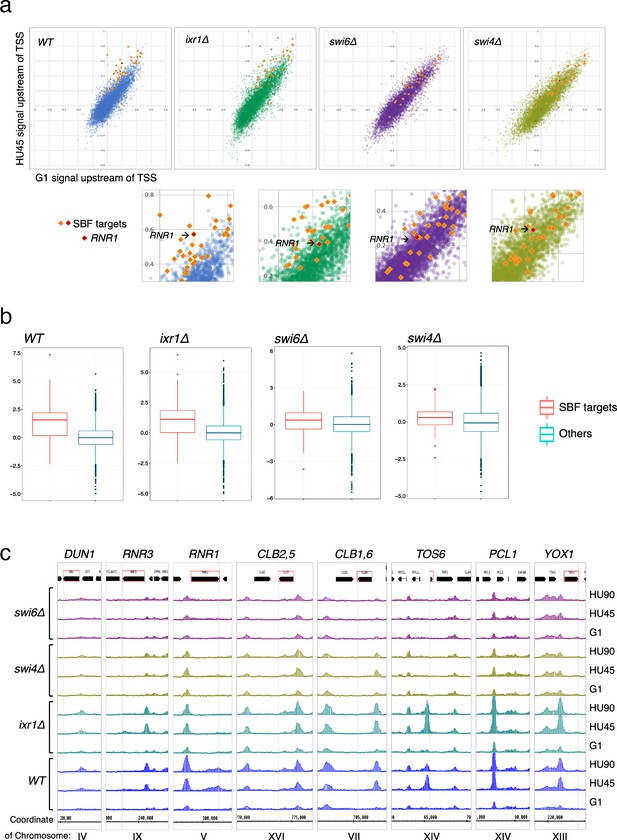
SBF plays a major role in the localization of Rad53 to the promoters of its target genes under replication stress.
(a) Top panels: Scatter plots compare Rad53 ChIP-seq signals in G1 (x-axis) and HU45 (y-axis) at 500-bp intervals upstream of transcription start sites (TSSs) for all genes in WT, ixr1Δ, swi4Δ, and swi6Δ mutants. Signals for SBF targets found in the 435 top DB overlap are shown as orange or red diamond (RNR1 in red diamond). Signals for the rest of genes are shown as dots in color blue (WT), green (ixr1Δ), purple (swi6Δ), and light olive (swi4Δ). Bottom panels: Close-up for specific area from above panels. (b) Box plots showing Z-score distribution for DB residuals (G1 → HU45) for genes that are annotated as targets for both Swi4 and Swi6 (SBF targets) and genes that are otherwise (Others) in WT, ixr1Δ, swi4Δ, and swi6Δ mutants (p value by Wilcoxon rank sum test: WT, 2.2e-16; ixr1Δ, 3.174e−12; swi4Δ, 0.001608; swi6Δ, 0.002857). (c) Coverage tracks showing distribution of Rad53 ChIP-seq signal near selected top DB genes in WT, ixr1Δ, swi4Δ, and swi6Δ mutants at stages of G1, HU45, and HU90.
In the ixr1Δ mutant, the Z-score distribution of Rad53 DB residual (G1 → HU45) showed a deviation similar to the level in WT (Figure 8b), and the Rad53 ChIP-seq signal for the majority of these SBF targets remained deviated from the general trend in the scatter plot. However, one clear exception was the RNR1 gene, indicated in the close-up plots (Figure 8a, lower panels), whose position collapsed in all three mutants, consistent with RNR1 being a target of Ixr1, SBF, and MBF (de Bruin et al., 2006; Tsaponina et al., 2011).
Discussion
Previous studies have implicated Rad53 kinase in regulation of gene expression through direct interaction with various transcription regulators (Jaehnig et al., 2013; Bastos de Oliveira et al., 2012; Sidorova and Breeden, 2003; Travesa et al., 2012). Unexpectedly, we found that Rad53 bound to about 20% of the gene promoters in the yeast genome. These genes encode proteins with diverse functions, including various aspects of cell cycle, metabolism, protein modification, ion transport, cell wall organization, and cell growth. There is a general trend of increasing Rad53 level during the G1- to S-phase transition in the presence of HU (Figures 2a and 3a). Remarkably, Rad53 binding at promoters for genes such as RNR1, RNR3, and TOS6 increases substantially beyond the general trend (Figures 2c and 3c). In contrast, Rad53 binding decreased at promoters of genes involved in response to mating pheromone as cells exited from α-factor-induced G1 arrest into the cell division cycle. The prevalent and dynamic changes in Rad53 promoter-bound levels in cells replicating in HU did not necessarily depend on checkpoint signaling activated by HU, for example, at genes like PCL1 and YOX1. But in some cases, such as RNR1, the increase in Rad53 levels was reduced in checkpoint mutants (Figure 2—figure supplement 4). Furthermore, the Mrc1 checkpoint mediator, Mec1 sensor kinase, and kinase activity of Rad53 itself are not absolutely required for the recruitment of Rad53 to gene promoters (Figure 1b and Figure 4—figure supplement 1). The binding of Rad53 to numerous promoters in the yeast genome suggests a previously unappreciated level of transcription regulation that is coordinated with the many cellular processes, such as normal DNA replication, and induced cellular stresses to which Rad53 responds.
The conditions employed in this study, cell cycle entry in the presence of HU, may determine the nature of the genes that display dynamic binding of Rad53 to gene promoters. It is known that Rad53 phosphorylates transcription factors such as the SBF and MBF subunit Swi6 and the MBF corepressor Nrm1 (Bastos de Oliveira et al., 2012; Sidorova and Breeden, 2003; Travesa et al., 2012) and that Irx1 controls transcription of RNR1 (Tsaponina et al., 2011). Removal of Swi4, Swi6 or Ixr1 reduced, and in some cases eliminated Rad53 binding to promoters of genes controlled by these transcription factors. We also found Rad53 bound to the NRM1 promoter, which is also in the top DB group, suggesting an additional regulation of cell cycle-dependent transcription control by Rad53. Rad53 also bound to promoters of genes encoding histones and all histone genes were upregulated DEGs in the HU45 (rad53K227A vs. WT) comparison (Figure 6—figure supplement 1), suggesting that, in addition to its known role in histone degradation (Gunjan and Verreault, 2003), Rad53 may control histone level through gene expression. This is consistent with previous findings that Rad53 targets Yta7 (Smolka et al., 2006), which interacts with FACT to regulate histone gene expression and inhibits Spt21NPAT-regulated histone genes expression (Bruhn et al., 2020; Gradolatto et al., 2008). In the absence of Rad53 protein, histone levels become elevated, causing global effects on gene expression (Bruhn et al., 2020; Tsaponina et al., 2011).
Our data are consistent with the possibility that the Rad53 protein contributes to the transcriptional regulation as a structural component by binding directly to promoters or transcriptions factors bound to these promoters, as previously suggested for several MAP kinases (Alepuz et al., 2001; Kim et al., 2008; Sanz et al., 2018). Like the stress-induced kinase Hog1, Rad53 binding to promoters may be dynamic in other stress conditions, which is under investigation. A major unanswered question is how Rad53 binds to so many diverse promoter sites. We suggest that it may bind DNA directly or to a common factor that is present at the promoters of Rad53-bound genes. Alternatively, certain chromatin features common to these promoters may facilitate Rad53 recruitment. It remains possible that, in addition to transcriptional regulation, Rad53 may be recruited to promoter region preemptively to ensure integrity of vulnerable chromatin associated with active transcription. Pre-targeting repair complexes to open chromatin where they are poised for lesion recognition and repair has been reported in human cells (Bacolla et al., 2021).
In cells, the same DNA template is used for both replication and transcription, potentially creating conflicts between replication and transcription that can lead to detrimental effects on genome stability and cell viability (Hamperl and Cimprich, 2016). Thus, it is important that cells employ mechanisms to avoid, tolerate and resolve such conflicts. It is known that late-replicating genes are tethered to the nuclear pore complexes in the nuclear periphery and checkpoint signaling, including Rad53 kinase, is required for preventing topological impediments for replication fork progression (Bermejo et al., 2011; Hamperl and Cimprich, 2016). Moreover, during normal replication, Mec1 may locally activate Rad53 to deal with difficult to replicate loci or regions of replication–transcription conflict without triggering full blown checkpoint activation (Bastos de Oliveira et al., 2015).
Eukaryotic cells initiate DNA synthesis in a temporally controlled manner from multiple replication origins to ensure efficient duplication of the genome (Bell and Labib, 2016; Renard-Guillet et al., 2014). One of the main consequences of checkpoint activation is inhibition of late origin firing (Tercero et al., 2003). However, in the checkpoint mutants, these late origins become active and we found that Rad53 was recruited to the body of origin-proximal genes. Concomitantly, gene expression of these genes was reduced, perhaps mediated by recruitment of Rad53. While checkpoint signaling pathways are known to regulate gene expression, it is possible that untimely activation of the late origins results in a replication–transcription conflict and contribute to downregulation of genes in these checkpoint mutants. We suggest that the normal temporal order of replication of genes in the genome throughout S-phase, and possibly the order of transcription of genes, have evolved to prevent conflicts between replication and transcription, which is particularly important in a gene dense genome such as S. cerevisiae.
Materials and methods
Reagent type (species) or resource | Designation | Source or reference | Identifiers | Additional information |
---|---|---|---|---|
Strain, strain background (Saccharomyces cerevisiae) | YS2571 | doi:10.1073/pnas.1404063111 | MATa bar1Δ::TRP1 URA3::BrdU-Inc ade2-1 can1-100 his3-11,–15 leu2-3,112 trp1-1 ura3-1 | |
Strain, strain background (Saccharomyces cerevisiae) | YS3110 | This paper | MATa rad53K227A::KanMX4 bar1Δ::TRP1 URA3::BrdU-Inc ade2-1 can1-100 his3-11,–15 leu2-3,112 trp1-1 ura3-1 | |
Strain, strain background (Saccharomyces cerevisiae) | YS3285 | This paper | MATa mrc1Δ::KanMX4 bar1Δ::TRP1 URA3::BrdU-Inc ade2-1 can1-100 his3-11,–15 leu2-3,112 trp1-1 ura3-1 | |
Strain, strain background (Saccharomyces cerevisiae) | YS3382 | This paper | MATa rad9Δ::HIS3 bar1Δ::TRP1 URA3::BrdU-Inc ade2-1 can1-100 his3-11,–15 leu2-3,112 trp1-1 ura3-1 | |
Strain, strain background (Saccharomyces cerevisiae) | YS3388 | This paper | MATa ixr1Δ::HIS3 bar1Δ::TRP1 URA3::BrdU-Inc ade2-1 can1-100 his3-11,–15 leu2-3,112 trp1-1 ura3-1 | |
Strain, strain background (Saccharomyces cerevisiae) | YS3401 | This paper | MATa swi4Δ::HIS3 bar1Δ::TRP1 URA3::BrdU-Inc ade2-1 can1-100 his3-11,–15 leu2-3,112 trp1-1 ura3-1 | |
Strain, strain background (Saccharomyces cerevisiae) | YS3406 | This paper | MATa swi6Δ::HIS3 bar1Δ::TRP1 URA3::BrdU-Inc ade2-1 can1-100 his3-11,–15 leu2-3,112 trp1-1 ura3-1 | |
Strain, strain background (Saccharomyces cerevisiae) | YS2828 | doi:10.1101/gr.195248.115 | MATa URA3::BrdU-Inc ade2-1 can1-100 his3-11,–15 leu2-3,112 trp1-1 ura3-1 | |
Strain, strain background (Saccharomyces cerevisiae) | YS3066 | doi:10.1101/gr.195248.116 | MATa sml1Δ::HIS3 URA3::BrdU-Inc ade2-1 can1-100 his3-11,–15 leu2-3,112 trp1-1 ura3-1 | |
Strain, strain background (Saccharomyces cerevisiae) | YS3075 | doi:10.1101/gr.195248.117 | MATa mec1Δ::TRP1 sml1Δ::HIS3 URA3::BrdU-Inc ade2-1 can1-100 his3-11,–15 leu2-3,112 trp1-1 ura3-1 | |
Strain, strain background (Saccharomyces cerevisiae) | YS3077 | doi:10.1101/gr.195248.118 | MATa rad53∆::KanMX sml1Δ::HIS3 URA3::BrdU-Inc ade2-1 can1-100 his3-11,–15 leu2-3,112 trp1-1 ura3-1 | |
Antibody | Anti-Rad53 antibody | Abcam | Cat# ab104232, RRID:AB_2687603 | |
Antibody | Anti-Histone H2A (phospho S129) antibody | Abcam | Cat# ab15083, RRID:AB_301630 | |
Antibody | Anti-Cdc45 antibody (polyclonal CS1485) | doi:10.1016/j.molcel.2006.07.033 | ||
Antibody | Anti-Orc6 antibody (monoclonal SB49) | Other | Stillman lab | |
Antibody | Anti Sml1 | Suppressor of Mec1 lethality antibody | Agrisera | Cat# AS10 847 | |
Peptide, recombinant protein | α-Factor | Other | WHWLQLKPGQPMY | |
Commercial assay or kit | TruSeq ChIP Library Preparation Kit | Illumina | Cat# IP-202-1012, IP-202-1024 | |
Commercial assay or kit | TruSeq stranded mRNA library preparation kit | Illumina | Cat# 20020594 | |
Commercial assay or kit | MinElute PCR purification kit | Qiagen | Cat# 28004 | |
Chemical compound, drug | Hydroxyurea | Sigma | H8627-25G | |
Software, algorithm | GraphPad Prism 9 | GraphPad | RRID:SCR_002798 | |
Software, algorithm | Bowtie | doi:10.1002/0471250953.bi1107s32 | RRID:SCR_005476 | |
Software, algorithm | bamCoverage | doi:10.1093/nar/gku365 | ||
Software, algorithm | STAR | doi:10.1093/bioinformatics/bts635 | ||
Software, algorithm | MACS2 | RRID:SCR_013291 | ||
Software, algorithm | ChIPpeakAnno | RRID:SCR_012828 | ||
Software, algorithm | DescTools | Andri Signorell et mult. al. (2021) | DescTools: Tools for descriptive statistics. R package version 0.99.41, 598 https://cran.r-project.org/package=DescTools |
Yeast strains and methods
Request a detailed protocolYeast strains generated in this study were derived from W303-1a (MATa ade2-1 can1-100 his3-11,15 leu2-3,112 trp1-1 ura3-1) and are described in Table 1. All the yeast strains used for the whole-genome DNA replication profile analyses have a copy of the BrdU-Inc cassette inserted into the URA3 locus (Viggiani and Aparicio, 2006). For G1 arrest of bar1Δ strains, exponentially growing yeast cells (~107 cell/ml) in YPD were synchronized in G1 with 25 ng/ml of α-factor for 150 min at 30°C. For G1 arrest of BAR1 strains, exponentially growing cells were grown in normal YPD, then transferred into YPD (pH 3.9), grown to ~107 cell/ml, and then synchronized in G1 with three doses of α-factor at 2 µg/ml at 0-, 50-, and 100 min time point at 30°C. Cells were collected at 150 min for release. To release from G1 arrest, cells were collected by filtration and promptly washed twice on the filter using one culture volume of H2O and then resuspended into YPD medium containing 0.2 mg/ml pronase E (Sigma).
Yeast strains used in this study.
Strain | Genotype | Source |
---|---|---|
YS2571 | MATa bar1Δ::TRP1 URA3::BrdU-Inc ade2-1 can1-100 his3-11,–15 leu2-3,112 trp1-1 ura3-1 | Sheu et al., 2014 |
YS3110 | MATa rad53K227A::KanMX4 bar1Δ::TRP1 URA3::BrdU-Inc ade2-1 can1-100 his3-11,–15 leu2-3,112 trp1-1 ura3-1 | This study |
YS3285 | MATa mrc1Δ::KanMX4 bar1Δ::TRP1 URA3::BrdU-Inc ade2-1 can1-100 his3-11,–15 leu2-3,112 trp1-1 ura3-1 | This study |
YS3382 | MATa rad9Δ::HIS3 bar1Δ::TRP1 URA3::BrdU-Inc ade2-1 can1-100 his3-11,–15 leu2-3,112 trp1-1 ura3-1 | This study |
YS3388 | MATa ixr1Δ::HIS3 bar1Δ::TRP1 URA3::BrdU-Inc ade2-1 can1-100 his3-11,–15 leu2-3,112 trp1-1 ura3-1 | This study |
YS3401 | MATa swi4Δ::HIS3 bar1Δ::TRP1 URA3::BrdU-Inc ade2-1 can1-100 his3-11,–15 leu2-3,112 trp1-1 ura3-1 | This study |
YS3406 | MATa swi6Δ::HIS3 bar1Δ::TRP1 URA3::BrdU-Inc ade2-1 can1-100 his3-11,–15 leu2-3,112 trp1-1 ura3-1 | This study |
YS2828 | MATa URA3::BrdU-Inc ade2-1 can1-100 his3-11,–15 leu2-3,112 trp1-1 ura3-1 | Sheu et al., 2016 |
YS3066 | MATa sml1Δ::HIS3 URA3::BrdU-Inc ade2-1 can1-100 his3-11,–15 leu2-3,112 trp1-1 ura3-1 | Sheu et al., 2016 |
YS3075 | MATa mec1Δ::TRP1 sml1Δ::HIS3 URA3::BrdU-Inc ade2-1 can1-100 his3-11,–15 leu2-3,112 trp1-1 ura3-1 | Sheu et al., 2016 |
YS3077 | MATa rad53∆::KanMX sml1Δ::HIS3 URA3::BrdU-Inc ade2-1 can1-100 his3-11,–15 leu2-3,112 trp1-1 ura3-1 | Sheu et al., 2016 |
Protein sample preparation and immunoblot analysis
Request a detailed protocolTCA extraction of yeast proteins was as described previously (Sheu et al., 2014). For immunoblot analysis, protein samples were fractionated by sodium dodecyl sulfate–polyacrylamide gel electrophoresis (SDS–PAGE) and transferred to a nitrocellulose membrane. Immunoblot analyses for Orc6 (SB49), Rad53 (ab104232, Abcam), γ-H2A (ab15083, Abcam), and Sml1 (AS10 847, Agrisera) were performed as described (Sheu et al., 2016; Sheu et al., 2014).
Isolation and preparation of DNA for whole-genome replication profile analysis
Request a detailed protocolModified protocol based on previously described (Sheu et al., 2016; Sheu et al., 2014). Briefly, yeast cells were synchronized in G1 with α-factor and released into medium containing 0.2 mg/ml pronase E, 0.5 mM 5-ethynyl-2′-deoxyuridine (EdU) with or without addition of 200 mM HU as indicated in the main text. At the indicated time point, cells were collected for preparation of genomic DNA. The genomic DNA were fragmented, biotinylated, and then purified. Libraries for Illumina sequencing were constructed using TruSeq ChIP Library Preparation Kit (Illumina). Libraries were pooled and submitted for 50-bp paired-end sequencing.
Sample preparation for ChIP-seq
Request a detailed protocolChromatin immunoprecipitation (ChIP) was performed as described (Behrouzi et al., 2016) with modification. About 109 synchronized yeast cells were fixed with 1% formaldehyde for 15min at room temperature (RT), then quenched with 130mM glycine for 5min at RT, harvested by centrifugation, washed twice with tris-buffered saline (50mM Tris–HCl pH 7.6, 150mM NaCl), and flash frozen. Cell pellets were resuspended in 600µl lysis buffer (50mM HEPES–KOH pH 7.5, 150mM NaCl, 1mM ethylenediaminetetraacetic acid, 1% Triton X-100, 0.1% Na-deoxycholate, 0.1% SDS, 1mM phenylmethylsulfonyl fluoride, protease inhibitor tablet (Roche)), and disrupted by bead beating using multi-tube vortex (Multi-Tube Vortexer, Baxter Scientific Products) for 12–15 cycles of 30s vortex at maximum intensity. Cell extracts were collected and sonicated using Bioruptor (UCD-200, Diagenode) for 38 cycles of pulse for 30s ‘ON’, 30s ‘OFF’ at amplitude setting High (H). The extract was centrifuged for 5min at 14,000rpm. The soluble chromatin was used for IP.
Antibodies against Cdc45 (CS1485, this lab Sheu and Stillman, 2006), Rad53 (ab104232, Abcam), γ-H2A (ab15083, Abcam) was preincubated with 1:1 mixture of washed Dynabeads Protein A and G (1002D and 1004D, Invitrogen) for more than 30min at RT and washed twice with lysis buffer to remove unbound antibodies. For each immunoprecipitation, 80μl antibody-coupled Dynabeads was added to soluble chromatin. Samples were incubated overnight at 4°C with rotation, after which the beads were collected on magnetic stands, and washed three times with 1ml lysis buffer and once with 1ml Tris-EDTA (50 mM Tris.–HCl pH 8.0, 10 mM EDTA), and eluted with 250μl preheated buffer (50mM Tris–HCl pH 8.0, 10mM EDTA, 1% SDS) at 65°C for 15min. Immunoprecipitated samples were incubated overnight at 65°C to reverse crosslink, and treated with 50μg RNase A at 37°C for 1hr. Then 5μl proteinase K (Roche) in 20mg/ml stock was added and incubation was continued at 55°C for 1hr. Samples were purified using MinElute PCR purification kit (Qiagen). Libraries for Illumina sequencing were constructed using TruSeq ChIP Library Preparation Kit (IP-202-1012 and IP-202-1024, Illumina).
Sample preparation for RNA-seq
Request a detailed protocolAbout 2–3 × 108 flash-frozen yeast cells were resuspended in Trizol (cell pellet:Trizol = 1:10) and vortexed for 15 s and incubated at 25°C for 5 min. Subsequently, 200 μl chloroform was added per 1 ml of Trizol–cell suspension and samples were vortexed for 15 s, incubated at RT for 5 min and centrifuged to recover the aqueous layer. The RNA in the aqueous layer were further purified and concentrated using PureLink Column (12183018A, Invitrogen). The RNA was eluted in 50 µl and store at −20°C if not used immediately. Store at −80°C for long term. Paired-end RNA-seq libraries were prepared using TruSeq stranded mRNA library preparation kit (20020594, Illumina).
Generation of coverage tracks using the Galaxy platform
Request a detailed protocolFor visualization of read coverage in the Integrated Genome Browser (Freese et al., 2016), the coverage tracks were generated using the Galaxy platform maintained by the Bioinformatics Shared Resource (BSR) of Cold Spring Harbor Lab. The paired-end reads from each library were trimmed to 31 bases and mapped to sacCer3 genome using Bowtie (Langmead, 2010). The coverage track of mapped reads was then generated using bamCoverage (Ramírez et al., 2014) with normalization to 1× genome.
Definition of the origin types
Request a detailed protocolBased on the BamCoverage output for EdU signal in WT, rad53K227A, and mrc1Δ, we categorized 829 origins listed in the oriDB database (Siow et al., 2012). We define the early origins as the one whose signal at the first time point is larger than 2. The late origins are extracted from the rest of the origins if the average signal value at the later time point is larger than 2 in rad53K227A and mrc1Δ mutants. Among the 829 entries in oriDB, we defined 521 as active origins (with EdU signal in WT or checkpoint mutants rad53K227A and mrc1Δ), in which 256 was categorized as early origins (with EdU signal in WT) and 265 as late origins (with signal in checkpoint mutants but not in WT). The remaining 308 entries do not have significant signal under our condition and were deemed inactive origins.
Computational analysis of sequence data
Request a detailed protocolThe sequenced reads were trimmed by cutadapt with an option of ‘nextseq-trim’, then aligned by STAR (Dobin et al., 2013) in a paired-end mode to the sacCer3 genome masked at repetitive regions. The gene structure is referred from SGD reference genome annotation R64.1.1 as of October 2018. For RNA-seq quantification analysis, the total counts of aligned reads were computed for each gene by applying ‘GeneCounts’ mode. For ChIP-seq quantification analysis, the reads were mapped using the same pipeline. We also confirmed the mapped reads found in ChIP-seq data do not span a long range (the median span is from 169.5 to 329, 90% paired-end reads are aligned within less than 6000-bp window), suggesting that STAR spliced alignment do not affect the alignment results. Additionally, peak calling was done by MACS2 in a narrow peak mode. Distribution of Rad53 ChIP-seq peaks was computed using ChIPpeakAnno.
The Gini indexes were calculated from Lorenz curves using Rad53 ChIP-seq datasets and published ChIP-Seq data for Swi6 (SRX360900 <https://www.ncbi.nlm.nih.gov/sra/SRX360900%5baccn%5d>: GSM1241092: swi6 DMSO illumina; S. cerevisiae; ChIP-seq), using DescTools (Andri Signorell et mult. al. (2021). DescTools: Tools for descriptive statistics. R package version 0.99.41, https://cran.r-project.org/package=DescTools).
Gene expression analysis
Request a detailed protocolDEGs and their p values were computed for each pair of the cases by nbinomWaldTest after size factor normalization using DESeq2 (Love et al., 2014). Using the list of DEGs, GO and KEGG enrichment analyses were performed via Pathview library. ClusterProfiler was applied to visualize fold changes of DEGs in each KEGG pathway. Coexpression analysis of significant DEGs was further performed based on coexpression network constructed in CoCoCoNet (Lee et al., 2020). CoCoCoNet has established the coexpression matrix of Spearman’s correlation ranking based on 2690 samples downloaded from SRA database. We carried out clustering for the correlation matrix downloaded from CoCoCoNet (yeast_metaAggnet) by dynamicTreeCut in R (or hierarchical clustering) to obtain at most 10 clusters. The enrichment analysis for the gene set of each cluster was performed in the same way with RNA-seq analysis.
ChIP-seq signal normalization
Request a detailed protocolFor ChIP-seq signal normalization, two different methods were applied to different types of analysis. For ChIP-seq residual analysis, we used simple normalization. In this process, each case sample was compared with the corresponding control sample of DNA input to compute log2 fold changes within each 25-bp window reciprocally scaled by multiplying the total read counts of another sample. Then, the average of fold changes was computed for each duplicate. For ChIP-seq heatmap analysis, we employed the origin-aware normalization to account for the higher background around origin region as a result of DNA replication. In the origin-aware normalization, the same computation used in simple normalization, or log2 fold change with scaling by the total read count, is independently applied for the region proximal to the origins and others. For the heatmap presented in this paper, the origin-proximal region is defined as the region within 5000-bp upstream and downstream.
Heatmap analyses at origins and TSS
Request a detailed protocolAfter the average fold change computation and normalization from ChIP-seq signals, the signal strength is visualized around the target regions such as TSSs and replication origins are extracted using normalizeToMatrix function in EnrichedHeatmap (window size is 25 bp and average mode is w0). We ordered heatmaps to examine a different signal enrichment pattern for the characteristics of each origin or gene. For the heatmap row of each origin is ordered by the assigned replication timing for ChIP-seq signals around replication origins. The replication time for the origins are annotated with the replication timing data published previously (Yabuki et al., 2002). From the estimated replication time for each 1000-bp window, we extracted the closest window from the center of each replication origin and assigned it as the representative replication timing if their distance is no more than 5000 bp. Early and late origins groups are categorized according to the definition of the origin types using the replication profile data from this study. The final set of the replication origins used in the heatmap analysis are obtained after filtering out the replication origins overlapped with any of 238 hyper-ChIPable regions defined in the previous study (Teytelman et al., 2013). In total, 167 early and 231 late origins pass this filter and are used in the heatmaps analysis in this study. For heatmaps of the ChIP-seq signals around TSS, we ordered genes based on RNA-seq fold changes for all DEGs or per coexpression cluster of DEGs based on gene coexpression network constructed in CoCoCoNet (Lee et al., 2020).
ChIP-seq residual analysis
Request a detailed protocolTo detect the time-dependent increase or decrease of Rad53-binding signals, we first focused on the 500-bp window upstream from each TSS (defined as promoter region) and computed the sum of the fold change signals estimated for each 25-bp window scaled by the window size as an activity of Rad53 binding for each gene. The overall activity scores are varied for each time point probably because of the different Rad53 protein level or other batch-specific reasons. To adjust such sample-specific differences for a fair comparison, a linear regression is applied for the activity scores of all genes between G1 and other time points HU45 and HU90 using lm function in R. Then we selected top genes showing the deviated signals from the overall tendency according to the absolute residual values between the actual and predicted values, excluding the genes with signal value lower than −0.075 after scaling the maximal signal to 1. Top 1000 genes with the highest absolute residual values were selected from two independent experiments. The 435 DB genes identified in both experiments (435 top DB overlap) were selected for further analysis.
Data availability
Sequencing data have been deposited in Dryad (https://doi.org/10.5061/dryad.tx95x69xv). The scripts used in this study including RNA-seq, ChIP-seq, and co-expression analysis are available at https://github.com/carushi/yeast_coexp_analysis (copy archived at swh:1:rev:3c890db7960a7665c34490593196732d5c3ad789).
-
Dryad Digital RepositoryPrevalent and dynamic binding of the cell cycle checkpoint kinase Rad53 to gene promoters.https://doi.org/10.5061/dryad.tx95x69xv
-
NCBI Sequence Read ArchiveID SRX362242. Sir2 input for Highly Expressed are Vulnerable to Misleading ChIP Localization of Multiple Unrelated Proteins.
-
NCBI Gene Expression OmnibusID GSE51251. Widespread Misinterpretable ChIP-seq Bias in Yeast.
References
-
Star: ultrafast universal RNA-seq alignerBioinformatics 29:15–21.https://doi.org/10.1093/bioinformatics/bts635
-
Molecular basis of the essential s phase function of the rad53 checkpoint kinaseMolecular and Cellular Biology 33:3202–3213.https://doi.org/10.1128/MCB.00474-13
-
Aligning short sequencing reads with bowtieCurrent Protocols in Bioinformatics 11:Unit.https://doi.org/10.1002/0471250953.bi1107s32
-
The yeast DNA damage checkpoint kinase rad53 targets the exoribonuclease, xrn1G3: Genes, Genomes, Genetics 8:3931–3944.https://doi.org/10.1534/g3.118.200767
-
G(1)/S and G(2)/M cyclin-dependent kinase activities commit cells to death in the absence of the S-phase checkpointMolecular and Cellular Biology 32:4971–4985.https://doi.org/10.1128/MCB.00956-12
-
Signaling pathways of replication stress in yeastFEMS Yeast Research 17:fow101.https://doi.org/10.1093/femsyr/fow101
-
DeepTools: a flexible platform for exploring deep-sequencing dataNucleic Acids Research 42:W187–W191.https://doi.org/10.1093/nar/gku365
-
Temporal and spatial regulation of eukaryotic DNA replication: from regulated initiation to genome-scale timing programSeminars in Cell & Developmental Biology 30:110–120.https://doi.org/10.1016/j.semcdb.2014.04.014
-
The essential kinase ATR: ensuring faithful duplication of a challenging genomeNature Reviews. Molecular Cell Biology 18:622–636.https://doi.org/10.1038/nrm.2017.67
-
Slt2 MAPK association with chromatin is required for transcriptional activation of Rlm1 dependent genes upon cell wall stressBiochimica et Biophysica Acta. Gene Regulatory Mechanisms 1861:1029–1039.https://doi.org/10.1016/j.bbagrm.2018.09.005
-
Rad53 checkpoint kinase phosphorylation site preference identified in the swi6 protein of Saccharomyces cerevisiaeMolecular and Cellular Biology 23:3405–3416.https://doi.org/10.1128/MCB.23.10.3405-3416.2003
-
OriDB, the DNA replication origin database updated and extendedNucleic Acids Research 40:D682–D686.https://doi.org/10.1093/nar/gkr1091
-
An FHA domain-mediated protein interaction network of Rad53 reveals its role in polarized cell growthThe Journal of Cell Biology 175:743–753.https://doi.org/10.1083/jcb.200605081
Decision letter
-
Jessica K TylerSenior and Reviewing Editor; Weill Cornell Medicine, United States
-
Miles A PufallReviewer; Department of Biochemistry and Molecular Biology, University of Iowa Health Care, Iowa City, United States
In the interests of transparency, eLife publishes the most substantive revision requests and the accompanying author responses.
Decision letter after peer review:
[Editors’ note: the authors submitted for reconsideration following the decision after peer review. What follows is the decision letter after the first round of review.]
Thank you for submitting the paper "Prevalent and Dynamic Binding of the Cell Cycle Checkpoint Kinase Rad53 to Gene Promoters" for consideration by eLife. Your article has been reviewed by 3 peer reviewers, and the evaluation has been overseen by a Reviewing Editor and a Senior Editor. The following individual involved in review of your submission has agreed to reveal their identity: Miles A Pufall (Reviewer #2).
Comments to the Authors:
We are sorry to say that, after consultation with the reviewers, we have decided that this work will not be considered further for publication by eLife.
After discussing the manuscript, the reviewers and the reviewing editor concluded that while the paper reports a series of interesting observations related to Rad53 binding to gene promoters independent of checkpoint signaling, the reviewers found the main message of the paper to be unclear. The paper starts with an attempt to look at RAD53 binding to replicons, but finds binding of RAD53 to promoter sites in G1 and in the absence of replication stress. Despite this intriguing observation, the results are analyzed with respect to stressed replication. As a result, the paper does not address reasons or consequences of the observed phenotype other than transcription/replication collisions.
From a technical point of view, all reviewers were concerned with the lack of statistics to draw inferences regarding the central question: Does Rad53 coordinate both replication and transcription in response to stress, and do the mutants disrupt this coordination. While it is often useful, to note a trend that is worth exploring, where numbers are available the validity of these inferences must be drawn to strengthen the conclusions.
Reviewer #1:
Strength: Upon first reading of the abstract, there was some concern that the observed Rad53 ChIP-seq at promoters could be related to the "expression bias" previously reported in ChIP-seq data sets, where false peaks were observed at some highly-expressed genes, even for exogeneous non-DNA binding proteins. The authors' analyses, especially lines 229-246, alleviate this concern.
Oftentimes throughout the manuscript the authors mention trends in the data, but without providing actual numbers. E.g. on line 180: "we noticed many Rad53 peaks […] and many of these localized […]"; providing numbers would be helpful for readers to understand the scale of the observed trends. The authors refer to specific figures, but those figures do not contain any numbers either.
As another example, at line 198, the authors say "Additional genes show increased Rad53 binding […], but at other promoters Rad53 binding decreases during the same time course […]. However, at most genes Rad53 remains constant." Numbers would be helpful here. Also, how were the increases and decreases called? What statistical tests and cutoffs were used to make these calls?
Same questions for page 6, lines 216-217. And also line 221, "numerous gene promoters" – could this be expressed as a fraction of all gene promoters in yeast?
The authors used "residual analysis in WT" to identify "the top differentially binding (DB) genes" in each set. Why residual analysis and not an established tool for differential binding, such as DEseq? And how were the different experiments in each set combined? For example, the TP set has 3 transcription factor null mutants and one WT condition. Comparing each mutant to the WT will gives three sets of differentially bound gene promoters; how are these combined? Is the direction of the change always the same across the 3 mutants? If not, what is reported in Figure 4b? What exactly is the y-axis in this figure panel?
The authors continue to say "Many of these genes encode proteins involved in cell cycle progression and cell growth". How was this determined? Was a statistical analysis (like GSEA) performed?
The relevance of the comparison between Rad53 ChIP-seq and Swi6 ChIP-seq is not entire clear. Did the authors mean to illustrate that although Rad53 binds to promoters, its pattern of binding is different from that of transcription factors? I.e. Rad53 binds a large set of promoters, but the range of binding signals is generally narrow, while transcription factors tend to bind strongly but to fewer promoters? If this was the intended purpose of the analysis (presented at lines 221-227), why was Swi6 selected? Would other cell-cycle transcription factors also be relevant for this type of analysis?
The comparisons between Rad53 binding and gene expression (line 248-284) show a clear positive correlation, especially in certain gene subsets/clusters. Further analyses, focused on targets of SBF, MBF, Msn4 and Ste12, confirm these correlations on subsets of genes targeted by these transcription factors. But it is unclear, to this reviewer, whether the authors conclusion that "regulation by SBF appears to be responsible for the correlation between increased Rad53 binding at the promoter and up-regulation of these target genes" is truly supported by the data. The correlations and overlaps are compelling, this is true, but are these significant and do they point to causality?
In Figure 8b, the authors highlight a few SBF targets that show "significant deviation" from the global trend. (How what the significance assessed?) But these are just the SBF targets in the "Top DB" set, i.e. in differentially bound gene promoters. Since this is how they were selected, shouldn't we expect them to deviate from the global trend? It is not clear how this finding shows that "Rad53 signal changes at these genes depends on SBF". This section (lines 364-283) continues with some examples of specific genes and their Rad53 promoter binding chances. But it is not clear what the general conclusion is according to these data.
One aspect that makes the manuscript difficult to read is that there are many observations reported throughout the paper, but it is not always clear how they relate to the main message of the paper. E.g. line 132: "the replication fork collapse was more severe in the absence of Rad53 kinase compared to the absence of checkpoint signaling in the mrcD mutant". This is an interesting observation, but what is the relevance for the main message of the paper?
As another example, in the paragraph at line 60 (Introduction), the authors talk about Rfx1 and Ixr1 transcription factors, and the RNR genes. But the relevance of this is unclear at this point.
As another example, the section at pages 7-8, lines 286-327, discusses Rad53 binding at gene bodies, rather than promoters. This is an interesting finding, but it distracts from the main message of the paper.
Page 5: The authors separate their ChIP-seq experiments into two groups, or sets: the CP set (WT, Rad53 mutant, and mrc1D) and the TP set (ixr1D, swi4D, swi6D and WT). While the conditions in the CP set are the ones used throughout the paper, the motivation behind the TP set in unclear. How were these particular factors selected? And are the "WT" assays the same in the two groups? For the transcription factors, the authors say they are selected "based on the types of genes that bind Rad53", but no other details are provided.
How was differential gene expression performed? (Figure 5)
Line 343: what does "enrichment score" refer to here? For this analysis, Fisher's exact test seems to be the most appropriate test for assessing enrichment of the Swi4/Swi6 targets among the differentially expressed genes.
For scatterplot analyses, e.g. Figure 8, there is no statistical analysis of the observed correlations. What are the R2 values and the corresponding p-values for these correlations?
As mentioned above, the choice of transcription factor null mutants for the Rad53 ChIP-seq experiments is not clearly motivated. SBF (Swi6+Swi4) is definitely a relevant choice, but would this be the top choice in an unbiased analysis of transcription factor proteins? Data on transcription factor gene targets is available for many factors in yeast. An unbiased analysis across all transcription factors could be performed to determine which factor shows the most significant (Fisher's test?) overlap with gene promoters bound by Rad53. In such an analysis, would SBF be the top candidate? If so, this kind of analysis would significantly strengthen the manuscript. Similarly, how was Ixr1 chosen? Was it meant as a control?
Reviewer #2:
In the manuscript entitled "Prevalent and Dynamic Binding of the Cell Cycle Checkpoint Kinase Rad53 to Gene Promoters" the authors explore the possibility that occupancy by the replication machinery influences regulation of genes. To do this, the authors measure the occupancy of Rad53, a key signaling kinase for the DNA replication checkpoint (DRC) that binds to replication origins. The authors measure occupancy after putting cells under stress with hydroxyurea in both wild-type cells and in cells in which the DRC is impaired through a mutation in Rad53 and by deletion of Mrc1. With an intact DRC, early origins of replication are activated and are occupied by Rad53. When the DRC is impaired early origins of replication are also activated and are occupied by Rad53, but late origins of replication are activated and occupied as well, indicating a bypass of the DRC. In a key finding, it is also noted that Rad53 occupies regions that are upstream and downstream of a large number of genes, and that the expression of thousands of genes is altered when the DRC is impaired. A difference in Rad53 occupancy near genes often correlates with changes in the expression of these genes. In particular, it appears that an increase in Rad53 binding close to the transcription start site of genes, both upstream and downstream, is correlated with the reduced expression of these genes. A deeper analysis of SBF target genes related to cell cycle and mating-type switching shows a correlation between Rad53 occupancy and gene expression. This appears to show two roles for Rad53 occupancy: inhibition of transcription at some genes when checkpoints are impaired, but also potentially as a transcriptional activator at others. With these roles, the authors assert that Rad53 coordinates both replication and gene expression under replication stress.
There are two major strengths to this manuscript. The first is that transcription-replication conflicts are increasingly being realized as occurring in eukaryotes, and this manuscript provides an excellent example of when such conflicts might occur. The carefully chosen mutants that bypass DRC even under stress show the aberrant firing of late origins in the proximity of genes whose expression is impaired. This provides a relevant and useful system to study conflicts when replication and transcription are not coordinated. The second major strength of this manuscript is the thorough collection of rich data sets in this system. The correlation of origin activity, Rad53 occupancy, and gene expression in WT and mutant backgrounds before and after administration of HU to induce replication allows probing of correlations between aberrant responses to stress and gene expression. The authors provide useful analyses of these data and demonstrate that bypass-induced binding of Rad53 correlates with reduced gene expression at numerous genes. Thus, their main assertions are supported by the data.
The main overall weaknesses of the paper are that it is difficult to read with little effort spent to make the work accessible outside the field of replication stress, the findings are not always well connected to provide a convincing argument, the figure resolution often makes them unreadable and impossible to interpret, and some statistical analyses are either missing or inappropriate. The weaknesses are detailed below:
There is a widespread use of indefinite quantifiers (some, many, several) to make quantitative inferences. Such as:
Line 200: "most genes" remain constant. How may genes go up, down, and remain constant?
Line 221: "Visual inspection of the ChIP-seq peaks suggested that Rad53 bound to numerous gene promoters and TSSs throughout the genome." How many is numerous? What fraction of TSSs?
Line 305: "Overall, most of the down regulated genes in cluster 1 of this group are situated very close to active origins". Reporting numbers make this more convincing.
Line 316: "More down regulated genes are found when the nearby origins are active." Reporting numbers make this more convincing.
Virtually all of the SBF target analysis.
Without quantification and statistics these amount to anecdotal observations. A quantitative inference requires the exact counting of groups followed by a statistical test.
Comment 1: The introduction is confusing. Line 47: After describing DRC and DDC along with four different kinases, the final sentence states that "the signaling" promotes widespread gene expression changes. All signaling? Rad53 specifically? Line 50 says that Mac1 and Rad53 are essential for cell viability, but Line 52 states that kinase null mutants (presumably either kinase?) are extremely sick. Which is it, sick or dead (essential)? The next sentence states that under bypass conditions (not defined) that sml1- and rad53- cells exhibit a "more severe defect" than mec1- cells, and that implies a role for Rad53 beyond DRC. This is either contradictory to Rad53 being essential, or too much background is left out to understand this pathway under different conditions. In addition, the authors already state that Rad53 has a role in DDC, which is beyond its function in DRC. Later the authors do not do a good job connecting RNR to "upregulating dNTP pools". This introduction may be clear to those familiar with Rad53 already, but not for others including those interested in transcription regulation or other aspects of signaling.
As a smaller point, the authors claim in the last paragraph that Rad53 is localized to 20% of promoters suggesting a "global" role in coordinating the response. 20% is not global – it may indicate a multifaceted role in response but global is a very high bar.
Comment 2: Most of the intro talks about the central role of Mec1 in sensing stress – yet the authors chose to first explore mrc1- cells and their stress response. What's the rationale for choosing that and not mec1-?
Comment 3, Line 84. The title doesn't help the reader understand the result. Having more heading that emphasized the results might help the reader more.
Comment 4: Line 101. The accounting for early, late, and inactive origins is confusing in the text but explained better in the methods. In this accounting early is defined as active in WT and late is defined as active in the mutants but not wild type. However, in Figure 2 origins are ranked according to replication timing define elsewhere (Yabuki 2002). Why the difference in the categorization? Do the classifications match perfectly? If so, why not use the Yabuki classification for both and avoid confusion?
Comment 5, Line 105. The assertion is that rad53_K227A favors late origins over early. Figure 1b shows a scatter plot with signal as the y-axis. It appears that a statistical test has been done to presumably show a significant difference between E (early) and L (late). What test was done (not described in body or figure)? Is the test for the difference in signal? If for signal, it could be that there are strong late ORCs, but more early ORCs – which would be a different test. My reading of "favor" late ORCs would be that there is a greater number of late than early, and that signal is how active the favored ORCs are.
Comment 6, line 138. The meaning of "the status of replisomes" is not defined and potentially broad. Something more specific would be helpful.
Comment 7, Line 146. The evidence of "slower progression" of Cdc45 away from the origin in mrc1- mutants is not clear to me. Could the authors describe what they are seeing to help the reader? The heat maps in Figure 2 Supplement 1 are duplicated in Figure 2 b,d, and f. This is not necessary and could be (was) confusing. Further, the Replication TIme scales in Figures 2b,d, and f seem to overlap between early and late – which is odd.
Comment 8, Line 163 "In contrast, the Mrc1 is not strictly required to induce or maintain -H2A." is a conclusion that is drawn without describing the result. The result appears to be that gammaH2A deposition is strong in the mutant and persists even at late time points.
Comment 9: The speculation in paragraph lines 166-169 would fit better in the discussion.
Comment 10, line 173. "dispersed in late times" – there is only one late time, HU90.
Comment 11, line 183. The claim that Rad53 signal increases from HU45 to HU90 is not 100% clear from the plots, and requires some type of test. Diffbind is great for this. Also – "A similar pattern occurs at the RNR3 promoter" I don't see this labeled in Figure 3.
Comment 12, line 190. How is "upstream" of the promoter defined? 1kb? 10kb? I don't see it in the results or methods.
Comment 13, paragraph 190-200. This is a discussion of Rad53 binding near TSSs. The fractions reported are the fraction of Rad53 peaks near genes. This is interesting, but the abstract reports that Rad53 "coordinates…genome-wide transcription" and binds "20%" of promoters. The number of promoters occupied by Rad53 is not reported here and should be accounted for to make these claims.
Comment 14: Paragraph lines 202-210. I found this paragraph confusing. First, the description of residual analysis in the methods was confusing. Summing in 25bp windows over 500bp upstream of TSS seems an odd way to calculate enrichment within a region, corrected for counts. R packages such as DiffBind do this quite rigorously, handle replicates well, and report differentially enriched regions with p-values. Also, reporting the difference in enrichment in regions between conditions is confusing – why not just enriched or depleted occupancy? I also could not quite follow what was meant by "435 genes identified in both". Please clarify, as much of the manuscript relies on this classification.
Comment 15. Figure 3d is not described in the legend, nor how it was calculated in the methods. This could be a useful plot but needs to be described.
Comment 16. The clustering conclusions in paragraph lines 250-256 draw distinctions that are not evident. The paragraph states that there is little difference in samples in G1, yet rad53-mutant and mrc1- samples cluster together. The paragraph also states that different from G1, rad53-mutant, and mrc1- samples cluster together in HU45 and HU90. The repeats of each mutant cluster together, but are not even always in the same clade (HU90). This interpretation is a stretch.
Comment 17. Lines 267-273. I had to read up on co-expression analysis and clustering. It is not clear to me why co-expression analysis is more useful than simple k-means clustering to identify sets of similarly differentially expressed genes. Perhaps a few words on why this method was chosen.
Comment 18. Line 321: "Furthermore, the bias toward the down regulation is even stronger (>80%) when the nearby origin is in a head-on orientation towards the gene." What is the percent when co-directional? Is the difference significant? It looks close enough that discerning a difference might require a statistical test (Fisher's Exact).
Comment 19. I had a hard time following the analysis of SBF and MBF target genes (page 8). First, an "Enrichment Score" is reported for the inclusion of SBF genes in the DEG set. I don't know what an enrichment score is. A more useful score is whether the number of potential SBF target genes is more likely to be differentially regulated than another random transcriptional complex. This can be calculated using Fisher's exact test. Otherwise, reporting that 36 out of 81 is anecdotal (unless the enrichment score is explained better). Second, Figures 8a bottom were impossible to read, and it was not clear what conclusions were to be drawn from them. Lastly, in paragraph lines 367-382, an analysis of the enrichment near genes in SBF mutants was performed. It is asserted that there is a "significant" difference in the enrichment of SBF targets among Top DBs – how was significance calculated? Then, in a series of pictures (Figure 8b insets) that the position of some enrichment is collapsed in mutants. Is this just visual? Calculating the Z-scores for these points and showing that the Z-scores reduce significantly would be a straightforward way to determine that the occupancy collapses toward the average.
Comment 20, lines 439-440. "Our data is consistent with the possibility that the Rad53 kinase contributes to the transcription regulation as a structural component" I don't know what this means. The manuscript asserts that the activity of Rad53 matters – so what is meant by the assertion that it is a "structural component"?
Comment 21. For rigor and reproducibility, the processing code should be included, preferably with raw data, in a manner that can be validated by the reviewer with one or few commands.
Reviewer #3:
In this study the authors find that unexpectedly RAD53 is bound to promotor regions of 20% of all yeast genes. The authors use genome wide ChIP-seq and correlative analysis to gene expression. Because of its known function during replication stress, experiments were designed with replication stress perturbation. However, the authors found RAD53 is bound to the promotor regions in G1 and without stress, and independent of canonical checkpoint signaling, making an analysis of experiments with replication stress somewhat illogical in the context of the specific discovery. Moreover, it introduces multiple additional variables directly affecting gene expression. This challenges the strength of interpretations of the correlations made.
Generally the correlations between RAD53 promotor biding and gene expression (some genes are some not) appear to be low. It is also unclear from the manuscript what matrix the authors use to claim significance of a given correlation.
Moreover, alternative interpretations are not considered. The authors solely investigate RAD53 promotor binding for its correlative effects on gene expression. While the finding that RAD53 is bound to promotor sites in the absence of stress is very interesting and promises for a potential greater implication of repair proteins in the conservation of transcription, promotor site binding of repair proteins have been previously reported Specifically, NEIL1 is preloaded at promotor sites of evolutionary critical genes and shown to promote rapid and efficient repair of promotor sites for conversation of critical genes. Such alternatives interpretation are not considered.
The authors may want to consider discussing (Nucleic Acids Research, Volume 49, Issue 1, 11 January 2021, Pages 221-243,) in the context of their discovery.
The authors use a kinase dead RAD53 mutant and a checkpoint dead mutant strain to suggest that RAD53 promotor binding is independent of a stress response. Recruitment to chromatin has been shown to involve acetylation rather than phosphorylation, as seen for Neil1, thus could be considered as an alternative to phosphorylation as stress signals, and alternative RAD53 mutant strains could be considered.
https://doi.org/10.7554/eLife.84320.sa1Author response
[Editors’ note: the authors resubmitted a revised version of the paper for consideration. What follows is the authors’ response to the first round of review.]
Comments to the Authors:
We are sorry to say that, after consultation with the reviewers, we have decided that this work will not be considered further for publication by eLife.
After discussing the manuscript, the reviewers and the reviewing editor concluded that while the paper reports a series of interesting observations related to Rad53 binding to gene promoters independent of checkpoint signaling, the reviewers found the main message of the paper to be unclear. The paper starts with an attempt to look at RAD53 binding to replicons, but finds binding of RAD53 to promoter sites in G1 and in the absence of replication stress. Despite this intriguing observation, the results are analyzed with respect to stressed replication. As a result, the paper does not address reasons or consequences of the observed phenotype other than transcription/replication collisions.
From a technical point of view, all reviewers were concerned with the lack of statistics to draw inferences regarding the central question: Does Rad53 coordinate both replication and transcription in response to stress, and do the mutants disrupt this coordination. While it is often useful, to note a trend that is worth exploring, where numbers are available the validity of these inferences must be drawn to strengthen the conclusions.
Despite criticisms on the previous version of manuscript (previous manuscript) about the clarity of background information and about lacking quantitative inferences and statistics (all have now been addressed in the revised manuscript), it is apparent that reviewers found many aspects of this study valuable with interesting observations and rich datasets. Furthermore, reviewer 2 pointed out that we “provide useful analyses of these data and demonstrate that bypass-induced binding of Rad53 correlates with reduced gene expression at numerous genes. Thus, their main assertions are supported by the data..” And reviewer 1 pointed out that our analysis alleviate the concern [related to the "expression bias" previously reported in ChIP-seq datasets, where false peaks were observed at some highly-expressed genes…]
We have made several changes in the revised manuscript (revised manuscript) and believe that this work merits consideration for publication by eLife.
Changes we have made to the manuscript and responses to general points
1) The previous manuscript included ample amount of data and analysis around the replication aspect of the Rad53 functions as these results constituted as logical connection to the prior knowledge of this kinase. Nevertheless, upon further consideration, we realized this part of work, while interesting, has become somewhat a distraction from the unexpected new finding that Rad53 is also functioning at gene promoters. Thus, after careful consideration, we decided to reorganize the presentation and removed panels related to DNA replicaiton from the main figures, mainly Figures 1 and 2 in the previous manuscript. The revised manuscript started directly from the finding of Rad53 localizing to gene promoters in asynchronous cell populations. We believe such presentation would direct the focus more appropriately and make the main message clear. Importantly, the revised manuscript also made quantitative inferences and relevant statistics more explicitly, as recommended by reviewers, to strengthen the conclusions.
2) The reviewers found the previous manuscript hard to read, particularly for readers without specific background knowledge. We acknowledged, indeed, that many criticisms of the previous manuscript arose because of misunderstanding of our presentation. The revised manuscript explained the background more carefully and thus improved the readability for more general audience.
3) Reviewers raised questions about some of less-conventional analysis choices we used in this study. In particular, the use of residual analysis instead of a common tool DiffBind for identification of to identify differential binding of Rad53 to gene promoters. Below, we provide further explanation and evidence that our methods are consistent with, and more appropriate than the conventional one for analysis of our datasets.
Briefly, residual analysis extracted the top 1000 deviated genes as top differential binding (DB) genes from aggregated read coverage while DiffBind computed significant q-values from duplicated signal comparison. We compared the DB genes identified from the two methods using G1 and HU45 datasets in experiment CP and found that the DB genes showed reasonable correlation (Spearman r = 0.7278) and p-value < 2.2e-16 for overlap (both 608, DiffBind only 2254, residual only 392, others 3873). We considered reporting the analysis using DiffBind as suggested by the reviewers, but there are several issues with applying DiffBind for these data sets.
i) Residual analysis controls for input whereas DiffBind does not.
ii) More than 40 % of all genes ended up as DB genes from WT G1 to HU45 in the CP experiment in DiffBind. This likely reflected the increased Rad53 protein level in HU45 thus this method is not ideal to determine which gene promoters are preferentially regulated.
iii) We further checked the reproducibility of DB genes by applying DiffBind to datasets from two independent experiments CP and TF. There were only 81 reproducible DB genes. In contrast, there were 435 reproducible DB genes when the residual analyses were applied to the same datasets.
Thus, we decided to use residual analysis to identify DB genes for further analysis.
Reviewer #1:
Strength: Upon first reading of the abstract, there was some concern that the observed Rad53 ChIP-seq at promoters could be related to the "expression bias" previously reported in ChIP-seq data sets, where false peaks were observed at some highly-expressed genes, even for exogeneous non-DNA binding proteins. The authors' analyses, especially lines 229-246, alleviate this concern.
We maintained these analyses that the reviewer deemed a strength in the revised manuscript.
Oftentimes throughout the manuscript the authors mention trends in the data, but without providing actual numbers. E.g. on line 180: "we noticed many Rad53 peaks […] and many of these localized […]"; providing numbers would be helpful for readers to understand the scale of the observed trends. The authors refer to specific figures, but those figures do not contain any numbers either.
As another example, at line 198, the authors say "Additional genes show increased Rad53 binding […], but at other promoters Rad53 binding decreases during the same time course […]. However, at most genes Rad53 remains constant." Numbers would be helpful here. Also, how were the increases and decreases called? What statistical tests and cutoffs were used to make these calls?
Same questions for page 6, lines 216-217. And also line 221, "numerous gene promoters" – could this be expressed as a fraction of all gene promoters in yeast?
All issues raised in this paragraph are addressed in the context of revised manuscript. Specific points from this paragraph are addressed as two separate points.
1)Concerning points raised for line 180 and line 221 in the previous manuscript: Quantitative inferences for the Rad53 binding to gene promoters are presented in the first section “Rad53 is recruited to genomic loci other than replication origins in proliferating yeast cells”.
There we described analysis that established the claim that Rad53 binds to more than 20 % of all gene promoters in proliferating cells (new manuscript lines 89-112). Furthermore, analysis of ChIP-seq peaks from a synchronized population showed that ~90% of the Rad53 ChIP-seq peaks were found at gene promoters at all stages investigated (Figure 2b).
2) Concerning points raised in line 198 and 216-217 of the previous manuscript:
These are addressed in the Results section “Identification of genes with differential binding (DB genes) of Rad53 at promoters”. There we used residual analysis to identify genes with differential binding of Rad53 at their promoters. (see “Changes we have made to the manuscript and responses to general points” section above for rationale to use residual analysis). Text in 216-217 of the previous manuscript is related to previous figure 3a. The exact figure has been removed as our main focus is on how specific transcription factors, Swi4 and Swi6, impact recruitment of Rad53 to promoters. Nevertheless, the normalized coverage tracks for WT samples in different stages are presented in the revised Figure 2c and the related text in Results section “Binding of Rad53 to upstream TSS changes with the cell cycle stages”. The presentation in revised Figure 2c merely pointed out that, even by visual inspection, signals at promoters of genes such as RNR1, PCL1 and TOS6 appeared varied depending on the cell cycle stage (G1, HU45 and HU90). This observation was further confirmed by the result of residual analysis described in the later subsection “Identification of genes with differential binding (DB genes) of Rad53 at promoters”. That is, these three genes were among the top ranking deviations in the residual analysis of WT samples in both of the two independent datasets.
The authors used "residual analysis in WT" to identify "the top differentially binding (DB) genes" in each set. Why residual analysis and not an established tool for differential binding, such as DEseq? And how were the different experiments in each set combined? For example, the TP set has 3 transcription factor null mutants and one WT condition. Comparing each mutant to the WT will gives three sets of differentially bound gene promoters; how are these combined? Is the direction of the change always the same across the 3 mutants? If not, what is reported in Figure 4b? What exactly is the y-axis in this figure panel?
The authors continue to say "Many of these genes encode proteins involved in cell cycle progression and cell growth". How was this determined? Was a statistical analysis (like GSEA) performed?
Issues raised in this paragraph will be address in three parts.
1) Regarding the reviewer's question “Why residual analysis and not an established tool for differential binding, such as DEseq?”, we believe the reviewer means DiffBind tool instead of DEseq, which is designed to apply a DE-seq2 strategy for ChIP-seq data. To validate the applicability of DiffBind for the samples with varied DNA input, we detected the DB genes in WT from two independent experiments CP and TF using DiffBind. As discussed above, a problem of reproducibility was found for DiffBind results presumably because DiffBind does not control for the input and did not take into account the general trends. See above “Changes we have made to the manuscript and responses to general points” section above for rationale to use residual analysis.
2) We regret the insufficient explanation that led to the questioning by the reviewer “how were the different experiments in each set combined?”. We have modified the description in the revised manuscript and indicated more clearly the datasets used in the analysis in the Results section “Identification of genes with differential binding (DB genes) of Rad53 at promoters”. In the case of our residual analysis for identifying genes with differential binding of Rad53 at promoters, only datasets of WT samples were used. We did not combine different experiments. Rather, each experiment has its own replicates and was analyzed separately and only data of stage G1 and HU45 from the same experiments were used for comparison with each other. The result is shown in Figure 3 of the revised manuscript. The previous 4b (now revised Figure 3b) presented the 435 genes that were within the top 1000 ranking in both of the two independent analyses for datasets in experiment TF and datasets in experiment named CP. In response to reviewer’s specific point, the y-axis numbers in the corresponding figure panel are residuals from analysis of experiment TF and this is now stated in the figure legend in revised manuscript.
3)Among the 435 genes identified, there are genes that have well-documented functions in cell cycle, cell growth, etc. Thus, we merely point this out in the text. The statement is based on prior knowledge rather than the implicating bioinformatical analysis. We believe, in addition to hints from bioinformatics, it is equally justified to use the knowledge from the literatures for direction. After all, these are very well characterized genes.
The relevance of the comparison between Rad53 ChIP-seq and Swi6 ChIP-seq is not entire clear. Did the authors mean to illustrate that although Rad53 binds to promoters, its pattern of binding is different from that of transcription factors? I.e. Rad53 binds a large set of promoters, but the range of binding signals is generally narrow, while transcription factors tend to bind strongly but to fewer promoters? If this was the intended purpose of the analysis (presented at lines 221-227), why was Swi6 selected? Would other cell-cycle transcription factors also be relevant for this type of analysis?
The related data (in revised manuscript Figure 2d) is to show the low specificity of Rad53 binding sites compared with binding sites of Swi6. In other words, Rad53 has a higher coverage for many promoters while Swi6 shows substantially high coverage only for a limited number of promoters, as the reviewer pointed out. Thus, it is likely Rad53 would effect a wider range of genes than the Swi6 regulatory network. Swi6 was chosen for this analysis because in the work presented later in the manuscript, where we found that deletion of Swi6 affected Rad53 recruitment to promoters in a small subset of genes. Furthermore, Swi6 would affect two major cell-cycle transcription factors SBF and MBF. The comparison between Rad53 and Swi6 biding to gene promoters emphasizes the observation that Rad53 binds to many more genes than conventional transcription factors.
The comparisons between Rad53 binding and gene expression (line 248-284) show a clear positive correlation, especially in certain gene subsets/clusters. Further analyses, focused on targets of SBF, MBF, Msn4 and Ste12, confirm these correlations on subsets of genes targeted by these transcription factors. But it is unclear, to this reviewer, whether the authors conclusion that "regulation by SBF appears to be responsible for the correlation between increased Rad53 binding at the promoter and up-regulation of these target genes" is truly supported by the data. The correlations and overlaps are compelling, this is true, but are these significant and do they point to causality?
The issue raised here might be semantic. We agree with the reviewer that the correlation and overlaps do not demonstrate causality and we did not intend to claim such. We have now modified the statement to "regulation by SBF may be responsible for the correlation between increased Rad53 binding at the promoter and up-regulation of these target genes" to simply point out the SBF might have a role in increased Rad53 binding, in addition to its role in up-regulation of SBF targets. And this led us to the next investigation presented in Result section “SBF is a key factor for recruitment of Rad53 to the promoters of its target genes under replication stress condition”. There we presented the experiments to determine the contribution of various transcription regulators in recruitment of Rad53. And, indeed, mutation in SBF did affect Rad53 binding to the gene promoters of SBF targets (revised Figure 8).
In Figure 8b, the authors highlight a few SBF targets that show "significant deviation" from the global trend. (How what the significance assessed?) But these are just the SBF targets in the "Top DB" set, i.e. in differentially bound gene promoters. Since this is how they were selected, shouldn't we expect them to deviate from the global trend? It is not clear how this finding shows that "Rad53 signal changes at these genes depends on SBF". This section (lines 364-283) continues with some examples of specific genes and their Rad53 promoter binding chances. But it is not clear what the general conclusion is according to these data.
We modify the phrase "significant deviation" to “clear deviation” as it merely means that these SBF targets are in the 435 TopDB identified in residual analysis. And, as the reviewer pointed out, “since this is how they were selected”, we indeed expected “them to deviate from the global trend“ in the context of the WT datasets. Most importantly, however, is the fact that such deviation is no longer evident for these SBF targets when plotted using the signal data obtained from SBF mutants. This finding demonstrated that Rad53 signal changes at these gene promoters depends on SBF. This is the conclusion according to these data.
Furthermore, we added a new panel (suggested by reviewer #2), showing the Z-score distribution of Rad53 DB residual (G1→HU45) and found substantial deviation of SBF target genes from the rest of the genes in WT (revised Figure 8b), while in the swi4Δ and swi6Δ mutants, the deviation of the SBF targets was closer to other genes.
Note: in the revised manuscript, we use the term “general trend” instead of “global trend” to describe the overall increase of Rad53 ChIP signal in HU samples, likely due to increased Rad53 protein level in cells treated with HU (Figure 2 —figure supplement 1).
One aspect that makes the manuscript difficult to read is that there are many observations reported throughout the paper, but it is not always clear how they relate to the main message of the paper. E.g. line 132: "the replication fork collapse was more severe in the absence of Rad53 kinase compared to the absence of checkpoint signaling in the mrcD mutant". This is an interesting observation, but what is the relevance for the main message of the paper?
As another example, in the paragraph at line 60 (Introduction), the authors talk about Rfx1 and Ixr1 transcription factors, and the RNR genes. But the relevance of this is unclear at this point.
As another example, the section at pages 7-8, lines 286-327, discusses Rad53 binding at gene bodies, rather than promoters. This is an interesting finding, but it distracts from the main message of the paper.
The issues raised here about relevance of results and text at previous line 132… is addressed in the revised manuscript as we removed some of the data related to the replication function of Rad53. Please see above “Changes we have made to the manuscript and responses to general points”. We now focus on Rad53 binding to TSSs in many genes in the genome, which is a novel and very unexpected finding.
About previous line 60 in Introduction, information on RNR genes, Rfx1 repressor and Ixr1 are relevant to this study because they constitute some of the well-known responses in gene expression downstream of the Rad53 kinase. Furthermore, in this study, we also found that Rad53 bound to the RNR1 gene promoter and the binding was reduced in both ixr1Δ and SBF mutants (Figure 8).
About lines 286-327, related to the finding of Rad53 binding at gene bodies, we believe it is relevant as it suggests a role for Rad53 in gene regulation in the context of transcription- replication conflicts.
Page 5: The authors separate their ChIP-seq experiments into two groups, or sets: the CP set (WT, Rad53 mutant, and mrc1D) and the TP set (ixr1D, swi4D, swi6D and WT). While the conditions in the CP set are the ones used throughout the paper, the motivation behind the TP set in unclear. How were these particular factors selected? And are the "WT" assays the same in the two groups? For the transcription factors, the authors say they are selected "based on the types of genes that bind Rad53", but no other details are provided.
We regretted that the description and terms for CP and TP sets we used in the previous manuscript caused confusion. All the ChIP-seq experiments using synchronized cultures were done under the same conditions in this study. CP and TF simply referred to two independent experiments. In each independent experiment, WT samples from G1, HU45 and HU90 were included in parallel to samples from mutants at the same stages. The analysis related to what the reviewer was referring to in Page 5 (of previous manuscript) is the datasets used in residual analysis, in which only the datasets from WT samples were used (revised Figure 3). Detail of residual analysis done in this study is provided in the result section “Identification of genes with differential binding (DB genes) of Rad53 at promoters” and further detail in the Materials and methods section “ChIP-seq residual analysis”. Mutant datasets were not used in the analysis presented in this part of the study.
About the choice of transcription mutants in ChIP-seq experiment used for further analyses, we selected swi4Δ and swi6Δ mutants because we noticed SBF targets in both DEGs and Rad53 DB genes in the conditions analyzed (i.e., G1 vs HU45). See more detail in “Rad53 binding changes coincide with the changes in gene expression for targets of cell cycle regulators SBF and MBF” of the Result section. The ixr1Δ mutant was also examined because a previous investigation found that Ixr1 binds to the RNR1 promoter upon genotoxic stress and mediates Dun1- independent RNR1 gene regulation that requires Rad53, which exhibited dynamic binding at the RNR1 gene promoter in this study. These choices are explained in the revised paper, citing appropriate literature.
How was differential gene expression performed? (Figure 5)
Differential gene expression was analyzed in a pairwise comparison of RNA-seq data between genotypes (i.e., specific mutant vs WT) under the same conditions (i.e., stage G1, HU45 or HU90) or between different stages (e.g., G1 vs HU45) in the same genotype. See DEG type manifestation in revised Figure 4b (related to previous Figure 5). Detail of RNA-seq analysis is described in “Computational analysis of sequence data” and “Gene expression analysis” in the Materials and methods section.
Line 343: what does "enrichment score" refer to here? For this analysis, Fisher's exact test seems to be the most appropriate test for assessing enrichment of the Swi4/Swi6 targets among the differentially expressed genes.
We agreed with the point of the reviewer and have now presented Fisher's exact test for the enrichment of the transcription factor targets in the bottom panel of Figure 7a.
For scatterplot analyses, e.g. Figure 8, there is no statistical analysis of the observed correlations. What are the R2 values and the corresponding p-values for these correlations?
We agreed with the point of the reviewer and have now presented Spearman correlation r and the corresponding p-values under the scatterplots in Figure 7a (related to previous Figure 8a).
As mentioned above, the choice of transcription factor null mutants for the Rad53 ChIP-seq experiments is not clearly motivated. SBF (Swi6+Swi4) is definitely a relevant choice, but would this be the top choice in an unbiased analysis of transcription factor proteins? Data on transcription factor gene targets is available for many factors in yeast. An unbiased analysis across all transcription factors could be performed to determine which factor shows the most significant (Fisher's test?) overlap with gene promoters bound by Rad53. In such an analysis, would SBF be the top candidate? If so, this kind of analysis would significantly strengthen the manuscript. Similarly, how was Ixr1 chosen? Was it meant as a control?
The choice of the transcription factor null mutants for the Rad53 ChIP-seq experiments has been addressed in response to an earlier point by the same reviewer. We agreed with the reviewer that the choice was not unbiased but a candidate method based on prior knowledge.
While unbiased analysis across all transcription factors could be valuable, the data on transcription factor gene targets are compilations of various large scale studies and manually curated results and not filtered in the same way. Thus, the comparison between data of different transcription would be difficult. The choice of ixr1Δ mutant is addressed above to the same reviewer. In paragraph starting “About the choice of transcription mutants in ChIP-seq experiment….”
Reviewer #2:
In the manuscript entitled "Prevalent and Dynamic Binding of the Cell Cycle Checkpoint Kinase Rad53 to Gene Promoters" the authors explore the possibility that occupancy by the replication machinery influences regulation of genes. To do this, the authors measure the occupancy of Rad53, a key signaling kinase for the DNA replication checkpoint (DRC) that binds to replication origins. The authors measure occupancy after putting cells under stress with hydroxyurea in both wild-type cells and in cells in which the DRC is impaired through a mutation in Rad53 and by deletion of Mrc1. With an intact DRC, early origins of replication are activated and are occupied by Rad53. When the DRC is impaired early origins of replication are also activated and are occupied by Rad53, but late origins of replication are activated and occupied as well, indicating a bypass of the DRC. In a key finding, it is also noted that Rad53 occupies regions that are upstream and downstream of a large number of genes, and that the expression of thousands of genes is altered when the DRC is impaired. A difference in Rad53 occupancy near genes often correlates with changes in the expression of these genes. In particular, it appears that an increase in Rad53 binding close to the transcription start site of genes, both upstream and downstream, is correlated with the reduced expression of these genes. A deeper analysis of SBF target genes related to cell cycle and mating-type switching shows a correlation between Rad53 occupancy and gene expression. This appears to show two roles for Rad53 occupancy: inhibition of transcription at some genes when checkpoints are impaired, but also potentially as a transcriptional activator at others. With these roles, the authors assert that Rad53 coordinates both replication and gene expression under replication stress.
There are two major strengths to this manuscript. The first is that transcription-replication conflicts are increasingly being realized as occurring in eukaryotes, and this manuscript provides an excellent example of when such conflicts might occur. The carefully chosen mutants that bypass DRC even under stress show the aberrant firing of late origins in the proximity of genes whose expression is impaired. This provides a relevant and useful system to study conflicts when replication and transcription are not coordinated. The second major strength of this manuscript is the thorough collection of rich data sets in this system. The correlation of origin activity, Rad53 occupancy, and gene expression in WT and mutant backgrounds before and after administration of HU to induce replication allows probing of correlations between aberrant responses to stress and gene expression. The authors provide useful analyses of these data and demonstrate that bypass-induced binding of Rad53 correlates with reduced gene expression at numerous genes. Thus, their main assertions are supported by the data.
The main overall weaknesses of the paper are that it is difficult to read with little effort spent to make the work accessible outside the field of replication stress, the findings are not always well connected to provide a convincing argument, the figure resolution often makes them unreadable and impossible to interpret, and some statistical analyses are either missing or inappropriate. The weaknesses are detailed below:
There is a widespread use of indefinite quantifiers (some, many, several) to make quantitative inferences. Such as:
Line 200: "most genes" remain constant. How may genes go up, down, and remain constant?
Line 221: "Visual inspection of the ChIP-seq peaks suggested that Rad53 bound to numerous gene promoters and TSSs throughout the genome." How many is numerous? What fraction of TSSs?
Line 305: "Overall, most of the down regulated genes in cluster 1 of this group are situated very close to active origins". Reporting numbers make this more convincing.
Line 316: "More down regulated genes are found when the nearby origins are active." Reporting numbers make this more convincing.
Virtually all of the SBF target analysis.
Without quantification and statistics these amount to anecdotal observations. A quantitative inference requires the exact counting of groups followed by a statistical test.
We have addressed the overall weaknesses in the revised manuscript. The revised manuscript explains the background more carefully and thus improves the readability for a more general audience. In addition, we have presented relevant statistics more explicitly in the text and figures. Many thanks to the suggestion of the reviewer. See also above “Changes we have made to the manuscript and responses to general points”.
The numbers that the reviewer deem necessary in these series of examples, when possible, were indeed presented in the text or charts in the figures of the previous manuscript despite the use of the indefinite quantifiers (some, many, several) as the reviewer pointed out and we appreciated this. For example, as cited by the reviewer in the next comment, “…Rad53 is localized to 20% of promoters…” In the revised manuscript, we have made further improvement, in particular the presentation of statistical tests and we have also refrained from using the indefinite quantifiers.
More detailed response to comments of reviewer #2 below.
Comment 1: The introduction is confusing. Line 47: After describing DRC and DDC along with four different kinases, the final sentence states that "the signaling" promotes widespread gene expression changes. All signaling? Rad53 specifically? Line 50 says that Mac1 and Rad53 are essential for cell viability, but Line 52 states that kinase null mutants (presumably either kinase?) are extremely sick. Which is it, sick or dead (essential)? The next sentence states that under bypass conditions (not defined) that sml1- and rad53- cells exhibit a "more severe defect" than mec1- cells, and that implies a role for Rad53 beyond DRC. This is either contradictory to Rad53 being essential, or too much background is left out to understand this pathway under different conditions. In addition, the authors already state that Rad53 has a role in DDC, which is beyond its function in DRC. Later the authors do not do a good job connecting RNR to "upregulating dNTP pools". This introduction may be clear to those familiar with Rad53 already, but not for others including those interested in transcription regulation or other aspects of signaling.
As a smaller point, the authors claim in the last paragraph that Rad53 is localized to 20% of promoters suggesting a "global" role in coordinating the response. 20% is not global – it may indicate a multifaceted role in response but global is a very high bar.
We thank the reviewer for pointing out specifically the parts in the Introduction that were confusing for more general audience. In response, we have carefully rewrote the background section and made better explanation on connecting the functional relationship among RAD53, RNR1 and SML1 genes and their roles in regulating cellular dNTP level.
For the next smaller point, in the revised manuscript, instead of “global”, we used “multifaceted”, which indeed depicts more appropriately how we envision Rad53 would function. Great suggestion from the reviewer.
Comment 2: Most of the intro talks about the central role of Mec1 in sensing stress – yet the authors chose to first explore mrc1- cells and their stress response. What's the rationale for choosing that and not mec1-?
The focus of the study is Rad53. In the Introduction, Mec1 was mentioned as the upstream kinase of Rad53 and not really emphasized beyond this point. In the revised manuscript, we indeed started with a result showing that Mec1 is not required for the promoter binding of Rad53 (Figure 1b). We studied Mrc1 because of its specific function in the DRC branch that mediated the signal in response to HU stress. In the context of the revised manuscript, mrc1Δ mutant is a great tool for providing a window through which we could examine the effect of untimely activation of late origins on transcription of nearby genes.
Comment 3, Line 84. The title doesn't help the reader understand the result. Having more heading that emphasized the results might help the reader more.
We agree with the point of the reviewer. In the revised manuscript, we have used heading that emphasized the results for the subsection title.
Comment 4: Line 101. The accounting for early, late, and inactive origins is confusing in the text but explained better in the methods. In this accounting early is defined as active in WT and late is defined as active in the mutants but not wild type. However, in Figure 2 origins are ranked according to replication timing define elsewhere (Yabuki 2002). Why the difference in the categorization? Do the classifications match perfectly? If so, why not use the Yabuki classification for both and avoid confusion?
We define early, late, and inactive origins according to the activity of replication origins from our data on de novo DNA synthesis from origins. But this classification does not give us information of time order in continuum. Yabuki’s study, on the other hand, measured time order of chromosome replication rather than the activation time of each origin, though the replication time and origin activation time would largely correlate with each other. Furthermore, their data were measured under different growth conditions. We used their result as a guide to order the origins on the heatmaps for presentation purpose but did not use their data in specific analyses. Overall, the two classification agree with each other.
Comment 5, Line 105. The assertion is that rad53_K227A favors late origins over early. Figure 1b shows a scatter plot with signal as the y-axis. It appears that a statistical test has been done to presumably show a significant difference between E (early) and L (late). What test was done (not described in body or figure)? Is the test for the difference in signal? If for signal, it could be that there are strong late ORCs, but more early ORCs – which would be a different test. My reading of "favor" late ORCs would be that there is a greater number of late than early, and that signal is how active the favored ORCs are.
Mann-Whitney test has been done to show a significant difference in EdU signal (i.e. nucleotide incorporation) between E (early) and L (late) origins. The interpretation is there is more DNA synthesis from late origins observed than from early origins in this particular mutant up to the time when samples were collected. ORC is the Origin Recognition Complex that binds replication origins in a sequence-specific manner as the first step in DNA replication. But perhaps the reviewer meant origin here. We thank the reviewer for the question and will be more careful with the description when presenting this result in the future as this specific result related to DNA replication is no longer presented in the revised manuscript.
Comment 6, line 138. The meaning of "the status of replisomes" is not defined and potentially broad. Something more specific would be helpful.
Comment 7, Line 146. The evidence of "slower progression" of Cdc45 away from the origin in mrc1- mutants is not clear to me. Could the authors describe what they are seeing to help the reader? The heat maps in Figure 2 Supplement 1 are duplicated in Figure 2 b,d, and f. This is not necessary and could be (was) confusing. Further, the Replication TIme scales in Figures 2b,d, and f seem to overlap between early and late – which is odd.
Comment 8, Line 163 "In contrast, the Mrc1 is not strictly required to induce or maintain -H2A." is a conclusion that is drawn without describing the result. The result appears to be that gammaH2A deposition is strong in the mutant and persists even at late time points.
Comment 9: The speculation in paragraph lines 166-169 would fit better in the discussion.
Comment 10, line 173. "dispersed in late times" – there is only one late time, HU90.
Most of the result and discussion on replication is no longer presented in the revised manuscript. But we appreciate the reviewer’s careful reading and helpful suggestion and will use them as guidance for presentation of this result in the future. Furthermore, we would like to clarify the issue the reviewer raised about the presentation of heatmaps around origins. In the previous manuscript, the heatmaps in Figure 2 Supplement 1 and in Figure 2 b, d and f were not duplicated but different presentations of the same dataset. In the previous Figure 2 Supplement 1, the origins were ordered according to the replication timing determine in Yabuki’s study. (This presentation is now in Figure 2 Supplement 3 of the revised manuscript). In the previous Figure 2, we separated origins into two categories, i.e. early and late origins, according to our EdU incorporation data, and then ordered origins in each group according to Yabuki’s timing. As explained in the response to comment 4 (see above), the two classification agree with each other. However, disagreement can be anticipated in situations where passive replication from and adjacent early origins renders chromosomal DNA containing late origins becoming early-replicating regions.
Thus, it is expected to have some overlap in the time scales for early and late origins using Yabuki’s timing order.
Comment 11, line 183. The claim that Rad53 signal increases from HU45 to HU90 is not 100% clear from the plots, and requires some type of test. Diffbind is great for this. Also – "A similar pattern occurs at the RNR3 promoter" I don't see this labeled in Figure 3.
The reviewer is referring to the presentation of coverage tracks showing Rad53 binding signal increased from HU45 to HU90 at RNR1, TOS6, PCL1 gene promoters. RNR3 was also mentioned and its coverage tracks showing similar pattern were presented in a later figure. All the example genes mentioned here are DB genes identified by residual analysis (435 Top DB overlap). These genes also tested significant using DiffBind. However, we decided to use residual analysis instead of the DiffBind as the method to identify DB genes in this study. See “Changes we have made to the manuscript and responses to general points” section above for rationale to use residual analysis.
Labelling issue mentioned here no longer exists in the revised manuscript.
Comment 12, line 190. How is "upstream" of the promoter defined? 1kb? 10kb? I don't see it in the results or methods.
Here, we believe the reviewer was asking about how “upstream” of gene is defined in the pie charts showing distribution of Rad53 peaks in relation to genes. In the revised manuscript, we replaced these charts with distribution of aggregated peak numbers around TSS in Figure 2b. The analysis was computed using ChIPpeakAnno, which is cited in Materials and methods section. The peak annotation is obtained by annotatePeakInBatch function and the distribution of the distance to the transcription start sites (TSS) plotted using merged Rad53 ChIP-seq peaks from all three stages (G1, HU45 and HU90). See also related response to Comment 13.
Comment 13, paragraph 190-200. This is a discussion of Rad53 binding near TSSs. The fractions reported are the fraction of Rad53 peaks near genes. This is interesting, but the abstract reports that Rad53 "coordinates…genome-wide transcription" and binds "20%" of promoters. The number of promoters occupied by Rad53 is not reported here and should be accounted for to make these claims.
This is related to Comment 12, this analysis, as pointed out by the reviewer reported the fraction of Rad53 peaks near genes. But the number of promoters occupied by Rad53 (about 20 %) is a different question and is now analyzed by calculating empirical p-value for the ChIP- seq signal 500 bp upstream of TSS. (see “Rad53 is recruited to genomic loci other than replication origins in proliferating yeast cells” in result section of revised manuscript for detail)
Comment 14: Paragraph lines 202-210. I found this paragraph confusing. First, the description of residual analysis in the methods was confusing. Summing in 25bp windows over 500bp upstream of TSS seems an odd way to calculate enrichment within a region, corrected for counts. R packages such as DiffBind do this quite rigorously, handle replicates well, and report differentially enriched regions with p-values. Also, reporting the difference in enrichment in regions between conditions is confusing – why not just enriched or depleted occupancy? I also could not quite follow what was meant by "435 genes identified in both". Please clarify, as much of the manuscript relies on this classification.
We appreciate the reviewer’s comment about our presentation of analysis to identify genes with differential binding of Rad53 at their promoter. In the revised manuscript, the process of this analysis is presented in the Results section “Identification of genes with differential binding (DB genes) of Rad53 at promoters” and explained in more clarity. To detect differentially binding at gene promoters, we computed the read coverage for each 25 bp window due to the limitation of computational resource and time for deepTools. Note that this window size is a half of their default size 50 bp. We focused on the 500 bp window upstream from each TSS where gene promoters are expected to reside in S. cerevisiae and applied residual analysis to compare G1 and HU45 signals using datasets from two independent experiments in order to improve the reproducibility of our DB detection. We identified in each analysis the top 1000 ranking DB genes. Among them, 435 genes were found in both independent experiments (revised Figure 3a and b).
Comment 15. Figure 3d is not described in the legend, nor how it was calculated in the methods. This could be a useful plot but needs to be described.
Reference on the calculation of Gini indices and Lorenz curves is described in “Computational analysis of sequence data” of Materials and methods section. Lorenz curve is now in Figure 2d of the revised manuscript.
Comment 16. The clustering conclusions in paragraph lines 250-256 draw distinctions that are not evident. The paragraph states that there is little difference in samples in G1, yet rad53-mutant and mrc1- samples cluster together. The paragraph also states that different from G1, rad53-mutant, and mrc1- samples cluster together in HU45 and HU90. The repeats of each mutant cluster together, but are not even always in the same clade (HU90). This interpretation is a stretch.
This comment is about the hierarchical clustering of rank data analysis of RNA-seq datasets from cell samples of 4 different genotypes and each with 3 growth stages, now presented at Figure 4a of the revised manuscript. It is evident that G1, HU45 and HU90 samples each form a group, with HU45 and HU90 group together at higher level. Within the HU45 group, it is also clear that rad53K227A and mrc1Δ clustered together. We agree with reviewer’s observation on the clustering within HU90 group and have revised accordingly in the revised manuscript. Nevertheless, it is still evident that rad9Δ and WT are closer to each other than to rad53K227A and mrc1Δ.
Comment 17. Lines 267-273. I had to read up on co-expression analysis and clustering. It is not clear to me why co-expression analysis is more useful than simple k-means clustering to identify sets of similarly differentially expressed genes. Perhaps a few words on why this method was chosen.
We chose to use co-expression analysis because it took advantage of accumulated knowledge of sequence databases. This is mentioned in the revised manuscript. Moreover, co- expression analysis can suggest a de novo gene-gene relationship that is not annotated in GO. The k-means clustering is applicable to our datasets although it would not find meaningful clusters due to low variability (only two similar conditions are available, one is WT-rad9Δ and another is rad53K227A-mrc1Δ). The results will be also highly confounded with the stage- dependent difference rather than their functional similarities.
Comment 18. Line 321: "Furthermore, the bias toward the down regulation is even stronger (>80%) when the nearby origin is in a head-on orientation towards the gene." What is the percent when co-directional? Is the difference significant? It looks close enough that discerning a difference might require a statistical test (Fisher's Exact).
We appreciated the suggestion of the reviewer and have included Fisher’s exact test p- value in the text where the numbers were described. See “Checkpoint mutants cause down- regulation of gene expression near promiscuously active late origins” in Results section.
Comment 19. I had a hard time following the analysis of SBF and MBF target genes (page 8). First, an "Enrichment Score" is reported for the inclusion of SBF genes in the DEG set. I don't know what an enrichment score is. A more useful score is whether the number of potential SBF target genes is more likely to be differentially regulated than another random transcriptional complex. This can be calculated using Fisher's exact test. Otherwise, reporting that 36 out of 81 is anecdotal (unless the enrichment score is explained better). Second, Figures 8a bottom were impossible to read, and it was not clear what conclusions were to be drawn from them. Lastly, in paragraph lines 367-382, an analysis of the enrichment near genes in SBF mutants was performed. It is asserted that there is a "significant" difference in the enrichment of SBF targets among Top DBs – how was significance calculated? Then, in a series of pictures (Figure 8b insets) that the position of some enrichment is collapsed in mutants. Is this just visual? Calculating the Z-scores for these points and showing that the Z-scores reduce significantly would be a straightforward way to determine that the occupancy collapses toward the average.
We appreciated the reviewer’s points in this comment and have done the following in the revised manuscript:
1) Enrichment of transcription factor targets in the 236 DB/DEGs (defined in “The relationship between Rad53 promoter binding and gene expression” of the Results section) was presented with p-value of Fisher’s exact test underneath the scatter plots in Figure 7a, which also show the correlation between binding changes (DB residual) and expression changes (log2 FC) of the same transcription factor targets.
2) After careful consideration, we decided to remove the plots at bottom panel of previous Figure 8a as we believe that they merely provided more data without adding major insight.
3) Concerning the paragraph in lines 367-382 of previous manuscript: The analysis and scatter plots (Figure 8a of revised manuscript) presented in this section were comparing the ChIP-seq signal for all genes between G1 and HU45 for WT and transcription factor mutants. We did not say [“there is a "significant" difference in the enrichment of SBF targets among Top DBs] as stated by the reviewer. (Perhaps the reviewer is referring to the same section addressed above in points (1) and (2) of this comment?) Instead, we pointed out there that SBF targets in TOP DB showed “significant” deviation from the general trend in the WT data. The ChIP-seq signal for SBF targets were shown in colors different from the rest of genes in the scatter plots include ChIP-seq signal for all genes. The presentation is also visual and the lack of deviation is evident in the SBF mutants (swi6Δ and swi4Δ). In the revised manuscript, we use “substantial” deviation instead of “significant” deviation in this context. We agree that it is a good idea to calculate the Z-scores, as suggested by the reviewer, for the DB residuals and have now included the results in Figure 8b of the revised manuscript. Analysis of Z-score distribution for Rad53 DB residual (G1→HU45) also showed substantial deviation of SBF target genes from the rest of the genes in WT (Figure 8b), while in the swi4Δ and swi6Δ mutants, the deviation of the SBF targets was closer to other genes.
Comment 20, lines 439-440. "Our data is consistent with the possibility that the Rad53 kinase contributes to the transcription regulation as a structural component" I don't know what this means. The manuscript asserts that the activity of Rad53 matters – so what is meant by the assertion that it is a "structural component"?
The relevant text is revised as: “Our data is consistent with the possibility that the Rad53 …. as a structural component by binding directly to promoters or transcriptions factors bound to these promoters…” We think it is reasonable to make such speculation since Rad53 is pleiotropic and the importance of the kinase activity does not rule out a kinase-independent role for Rad53.
Comment 21. For rigor and reproducibility, the processing code should be included, preferably with raw data, in a manner that can be validated by the reviewer with one or few commands.
The scripts used in this study including RNA-seq, ChIP-seq, and co-expression analysis are available at https://github.com/carushi/yeast_coexp_analysis.
Reviewer #3:
In this study the authors find that unexpectedly RAD53 is bound to promotor regions of 20% of all yeast genes. The authors use genome wide ChIP-seq and correlative analysis to gene expression. Because of its known function during replication stress, experiments were designed with replication stress perturbation. However, the authors found RAD53 is bound to the promotor regions in G1 and without stress, and independent of canonical checkpoint signaling, making an analysis of experiments with replication stress somewhat illogical in the context of the specific discovery. Moreover, it introduces multiple additional variables directly affecting gene expression. This challenges the strength of interpretations of the correlations made.
We respectively disagree with the reviewers comments that the discovery is illogical. Science is advanced by experimenters making observations that are unexpected. We described the study in which we analyzed the localization of Rad53 in the genome using ChIP-seq in the original context of entry into S phase with a HU-induced DNA damage response. We did not expect that Rad53 bound to so many gene promoters in the DNA damage response and certainly did not expect that Rad53 would be bound to TSSs in G1 phase, but this is how discoveries are made. We did place emphasis in the revised paper on Rad53 binding to genes and particularly to TSS regions of genes rather than the origin binding observations to focus the paper more on Rad53 binding to promoters. We showed that in a number of cases, Rad53 binding is influenced by sequence specific transcription factors. This is not, in our view, illogical. Rather it is a novel discovery.
Generally the correlations between RAD53 promotor biding and gene expression (some genes are some not) appear to be low. It is also unclear from the manuscript what matrix the authors use to claim significance of a given correlation.
The reviewer pointed out that “the correlations between RAD53 promotor biding and gene expression (some genes are some not) appear to be low….”. However, the correlations, while not extremely high, are significant for specific subset of genes, catogorized by either co- expression clusters (Figure 5c) or target of specific transcription factors (Figure 7a). Results of statistical tests are included more clearly in the revised manuscript. Furthermore, we find it even more interesting that other co-expression clusters did not show clear correlations, suggesting the possibility these other subsets might be responding to different signals. In the follow up study we plan to investigate the effect of other type of stresses on Rad53 recruitment, dynamic binding and gene expression.
Moreover, alternative interpretations are not considered. The authors solely investigate RAD53 promotor binding for its correlative effects on gene expression. While the finding that RAD53 is bound to promotor sites in the absence of stress is very interesting and promises for a potential greater implication of repair proteins in the conservation of transcription, promotor site binding of repair proteins have been previously reported Specifically, NEIL1 is preloaded at promotor sites of evolutionary critical genes and shown to promote rapid and efficient repair of promotor sites for conversation of critical genes. Such alternatives interpretation are not considered.
The authors may want to consider discussing (Nucleic Acids Research, Volume 49, Issue 1, 11 January 2021, Pages 221-243,) in the context of their discovery.
The authors use a kinase dead RAD53 mutant and a checkpoint dead mutant strain to suggest that RAD53 promotor binding is independent of a stress response. Recruitment to chromatin has been shown to involve acetylation rather than phosphorylation, as seen for Neil1, thus could be considered as an alternative to phosphorylation as stress signals, and alternative RAD53 mutant strains could be considered.
We agreed with reviewer #3’s suggestion on alternative explanation of Rad53 recruitment to gene promoters. Thus, we have added discussion on the idea of preemptive recruitment of repair factors to potentially vulnerable chromatin regions and also included the recommended reference in the Discussion section. The reviewer also recommended studying alternative rad53 mutant strains, which we have indeed begun to incorporate in our follow up studies. We hope to report these new studies in a new manuscript.
For clarification, we suggested that recruitment of Rad53 to gene promoter does not require the kinase activity in cis and nor checkpoint signaling. However, in no way did we try to rule out the contribution of checkpoint signaling or Rad53 kinase activity on a subset of genes. Rather, we suggested that binding can be modulated by multitude of responses eliciated by the experimental condition, not solely the well-defined DRC checkpoint signaling pathway. And the best example is the RNR1 gene promoter, to which the Rad53 binding is affected by checkpoint response as well as cell cycle regulation (high-lighted in Figure 8a).
https://doi.org/10.7554/eLife.84320.sa2Article and author information
Author details
Funding
Uehara Memorial Foundation (Postdoctoral Fellowship)
- Risa Karakida Kawaguchi
National Institute of General Medical Sciences (GM45436)
- Bruce Stillman
National Cancer Institute (P30-CA045508)
- Bruce Stillman
National Library of Medicine (R01LM012736)
- Jesse Gillis
Goldring Family Foundation
- Bruce Stillman
The funders had no role in study design, data collection, and interpretation, or the decision to submit the work for publication.
Acknowledgements
This research was supported by NIH grants R01GM45436 and R01LM012736 and a gift from the Goldring Family Foundation. The Cold Spring Harbor Laboratory Cancer Center supported core research resources (P30-CA045508). RKK was supported by Uehara Memorial Foundation Postdoctoral Fellowship.
Ethics
This study used animals for antibody production and all studies were performed in strict accordance with the recommendations in the Guide for the Care and Use of Laboratory Animals of the National Institutes of Health. All of the animals were handled according to approved institutional animal care and use committee (IACUC) protocols (#20-17-14-11-8-05-02-18) of Cold Spring Harbor Laboratory.
Senior and Reviewing Editor
- Jessica K Tyler, Weill Cornell Medicine, United States
Reviewer
- Miles A Pufall, Department of Biochemistry and Molecular Biology, University of Iowa Health Care, Iowa City, United States
Version history
- Preprint posted: May 23, 2021 (view preprint)
- Received: October 20, 2022
- Accepted: November 29, 2022
- Accepted Manuscript published: December 15, 2022 (version 1)
- Version of Record published: December 28, 2022 (version 2)
Copyright
© 2022, Sheu et al.
This article is distributed under the terms of the Creative Commons Attribution License, which permits unrestricted use and redistribution provided that the original author and source are credited.
Metrics
-
- 771
- Page views
-
- 121
- Downloads
-
- 2
- Citations
Article citation count generated by polling the highest count across the following sources: PubMed Central, Crossref, Scopus.
Download links
Downloads (link to download the article as PDF)
Open citations (links to open the citations from this article in various online reference manager services)
Cite this article (links to download the citations from this article in formats compatible with various reference manager tools)
Further reading
-
- Chromosomes and Gene Expression
- Genetics and Genomics
Spermatogenesis in the Drosophila male germline proceeds through a unique transcriptional program controlled both by germline-specific transcription factors and by testis-specific versions of core transcriptional machinery. This program includes the activation of genes on the heterochromatic Y chromosome, and reduced transcription from the X chromosome, but how expression from these sex chromosomes is regulated has not been defined. To resolve this, we profiled active chromatin features in the testes from wildtype and meiotic arrest mutants and integrate this with single-cell gene expression data from the Fly Cell Atlas. These data assign the timing of promoter activation for genes with germline-enriched expression throughout spermatogenesis, and general alterations of promoter regulation in germline cells. By profiling both active RNA polymerase II and histone modifications in isolated spermatocytes, we detail widespread patterns associated with regulation of the sex chromosomes. Our results demonstrate that the X chromosome is not enriched for silencing histone modifications, implying that sex chromosome inactivation does not occur in the Drosophila male germline. Instead, a lack of dosage compensation in spermatocytes accounts for the reduced expression from this chromosome. Finally, profiling uncovers dramatic ubiquitinylation of histone H2A and lysine-16 acetylation of histone H4 across the Y chromosome in spermatocytes that may contribute to the activation of this heterochromatic chromosome.
-
- Chromosomes and Gene Expression
- Developmental Biology
Imaging experiments reveal the complex and dynamic nature of the transcriptional hubs associated with Notch signaling.